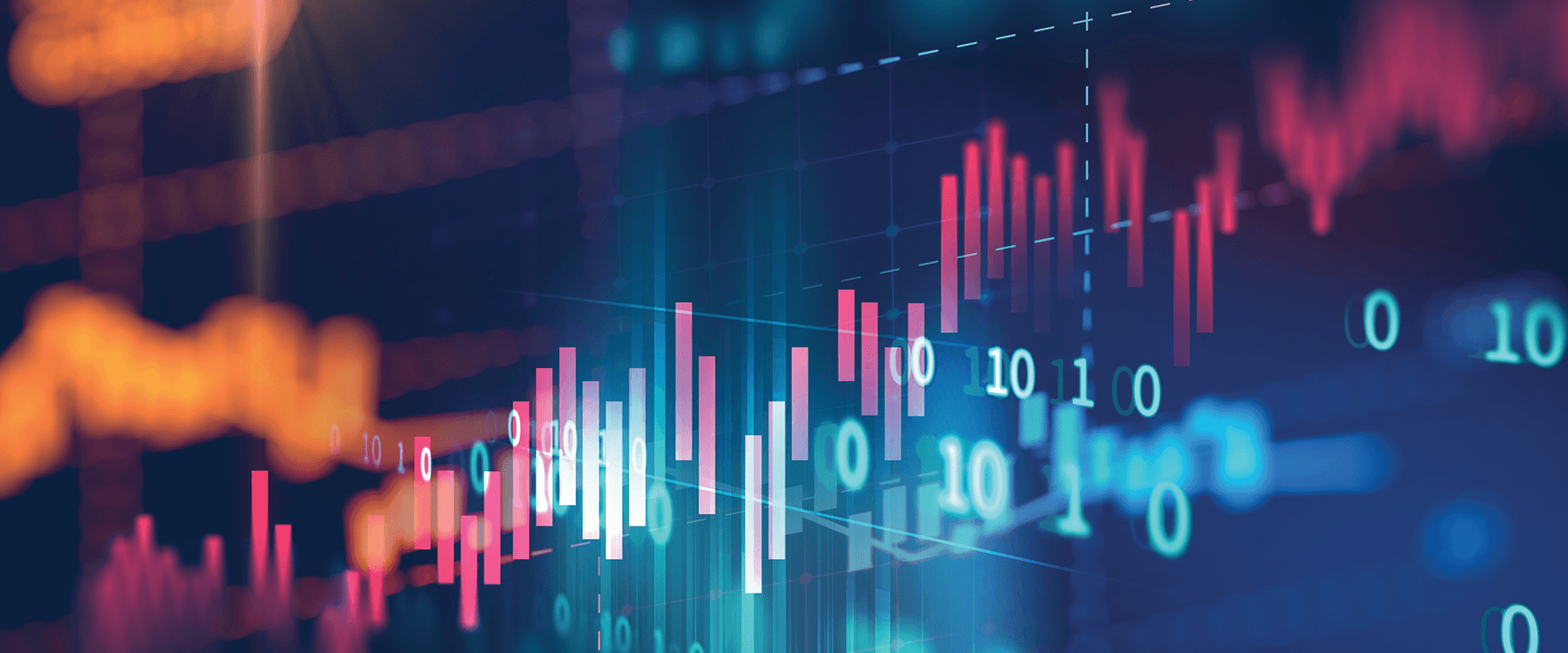
Don’t Just Rush to Adopt New Technologies — Plan a Path to Monetization by Following These Rules
- Volume XX, Issue 42
- Executive Insights
A wide range of transformative, interrelated and disruptive technologies have arrived, including AI, machine learning, natural language processing and blockchain.
However, a surge in irrational enthusiasm is driving businesses to develop technology solutions that might be too ambitious or not sufficiently connected to business needs.
As a result, businesses adopt technologies but fail to monetize them.
Our five-step framework makes it possible to plan and prioritize technologies — and identify projects that will be practical and generate value.
In addition, our six guiding principles can be used to apply the framework to technology planning specifications.
For businesses across virtually all sectors, a technology revolution is here — and fully accessible — right now.
A wide range of transformative, interrelated and disruptive technologies have arrived. They include artificial intelligence, machine learning (a subset beneath the AI umbrella that enables a computer to progressively learn a skill, process or decision rules); natural language processing (like Alexa or Siri); and blockchain (a secure, distributed ledger-keeping system for trusted identity and asset transactions, currently best known for cryptocurrency applications that can also be used for various forms of record keeping).
Used in combination, these technologies represent ways that companies can become more efficient, faster to market, more responsive to customers and more valuable. And in contrast to past technology adoption cycles, the technology environment is becoming more supportive than ever for businesses that want to take advantage. For example, thanks to cloud computing, remote web services, widespread broadband and ubiquitous cheap storage, it’s possible to rent (rather than buy) a technology infrastructure. Business leaders don’t need to own the technology or be entirely dependent on a large cadre of in-house technologists in order to get into the game.
But this ease of implementing new technologies has led to a surge in irrational technology enthusiasm that, coupled with competitive pressures, is driving businesses to invest the wrong way. Business leaders make two mistakes — closely related to each other, and both completely understandable given the competitive landscape and the prospect of a high upside, but damaging nevertheless: They proceed quickly, in a spirit of “Ready, fire, aim!” — jumping in too fast, without a clear sense of what problem they’re solving or what value they want to create. And in so doing, they fail to clearly define the use case and, thus, develop a technology solution that might be too ambitious, too long-term or just not sufficiently connected to a business need.
The result, and the core problem, is this: Businesses adopt technologies but fail to monetize them. What’s needed is a framework for thinking about technology adoption, one that provides guidance for establishing a path to monetization. In this Executive Insights, L.E.K. Consulting provides such a framework.
No question, technology is exciting. For a business technologist — or any technology enthusiast — there’s a thrill that goes along with the “reveal” of an ambitious technology project. Remember the sense of new possibilities you had when IBM’s Deep Blue learned chess and defeated grandmaster Garry Kasparov in 1997? Or more recently, when Google parent Alphabet created AlphaGo, a computer program that for the first time beat a professional player at the complex Chinese game of Go?
What lessons can we draw from projects like these? No doubt, the challenges they met and overcame were enormous. But what’s less obvious, yet highly relevant to the practical world of business technology, is that they were also very sharply defined. Winning at chess or Go is a very specific task — one that determines the focus and scope of the technology application, and the conditions and rules for success.
But most business technology projects aren’t nearly as sharply defined as “winning at chess” or “winning at Go.” The typical starting point for a business technology project is much more general: “We need an AI-based customer service solution,” or “We need to create a machine-learning system that can diagnose illnesses and present treatment options.”
These “asks” are ambitious and nebulous. And the result of that ambition is typically a project that is very broad in scope. The ambition and the broad scope, taken together, exacerbate the risk of failure — first of all, because there is no clear attempt to come to grips with practical questions: What will the project milestones be? What’s the timeline? What will the costs be? The ambition and scope of the project work against discipline and pragmatism. The broader the scope of the project, the longer the timeline until revenue is generated, the higher the development costs and the more risk there is of “mission creep,” where the project continually shifts and expands to meet evolving goals and loses its focus.
Technology projects need discipline to ensure they are practical, focused and monetizable. The way to establish this discipline is to ask questions that define the use case. Defining the use case is the key to transforming “pie in the sky” technology initiatives into practical solutions that lead to value — generating revenue, reducing costs, improving the efficacy of outcomes and enhancing competitive advantage.
The central question is not about technology first, but rather about the business challenge: What is the problem that needs to be solved, and where can technology be applied to solve that problem?
Framing the question is difficult, because technology projects can scale to solve different problems. But it is essential that the problem — not the potential of the technology — be the guiding factor.
Consider, for example, the challenges involved in creating an AI-based system for medical diagnoses (below):
The threshold for answering that question definitively is high, which makes for a long-term, high-cost project. If the AI system needs to determine with 100% accuracy whether or not the patient has cancer, then the tolerance for error is extremely low. Extensive work and experimentation must be done to optimize the algorithm. The Food and Drug Administration will likely want visibility into how the algorithm works, not to mention evidence of outcomes. Oncologists will resist it — they are being asked to surrender their judgment and experience to software.
This project has a long path to realization, with high development costs and extensive clinical and scientific collaborations, and many barriers to entry. But the threshold can be lowered — which results in a shorter-term, lower-cost project that will generate revenue sooner.
Now the bar is lower, and there is more tolerance for error. Relatively speaking, it will be easier, faster and less expensive to create an AI system that conducts an initial screening. The AI provides incremental value — it is efficient at flagging more cases and getting some patients into treatment sooner. The AI doesn’t say, “This patient has cancer.” It says, “This patient might be worth a second look.” This solution will likely need less regulatory review because it supports (and does not replace) medical professionals. And for that reason, there will be less resistance among oncologists, to the extent that there is value in knowing which patients are worth a closer look. The project, as defined by a less ambitious question, could be saving lives and generating revenue within a much shorter period of time.
Businesses need a systematic way to define the problem and harness the technology to the use case. We propose one example, which consists of the following:
Considering these five steps makes it possible to plan and prioritize technologies — and identify projects that will be practical and value-generating.
Variable No. 1: How attractive is the solution to the market? Does it solve a critical business issue, create value, produce a return on investment or open a competitive opportunity?
Variable No. 2: How feasible is the solution for technology vendors? Can it leverage current platform capabilities? Can it leverage ecosystem partnerships? What level of technological maturity has it reached?
Most technology solutions are seeking to correctly identify the “data lakes” (structured and unstructured data to be mined) as well as the technology tools (workflow automation, cognitive analytics, FDA algorithms, language and vision processing, etc.) to be used to leverage the data and collect value.
But few take the next step: defining the use case or the business need that the technology solution must meet. Most technology solutions are looking for the problem to answer.
Failure to connect the dots creates multiple risks: using the wrong data, using the wrong analytic approach and, most basically, asking the wrong questions. By first defining the use case (step 1) and then connecting the technology to the use case (step 2), business leaders can greatly increase the odds of success.
Total up the value of cost offset, labor cost savings, increased revenue, higher quality of product or service, and level of customer/client satisfaction.
For example, an AI technology solves a use case for a streaming service like Netflix by generating viewer recommendations — if it achieves the following:
In practice, technology projects aren’t evaluated in isolation. Companies generally have several under development. Technology projects should always be considered within the broader context of a platform. Which use cases can be bundled to create a sufficiently compelling value proposition for potential customers? Not all use cases will be immediately feasible (see step 5), but showing customers your overall technology road map and vision will encourage them to invest in foundational use cases.
Therefore, the next step is to create a matrix in which the highest priority goes to the projects that:
The result will be a rational platform strategy that captures immediate opportunities — and revenue — while still planning for the long-term future.
The technologies that are now arriving on the scene — and those that are soon to arrive — create an array of possibilities that are at once exciting and terrifying.
But neither excitement nor terror is the right response when it comes to business planning.
By applying our proposed example and focusing first on customers’ critical unmet needs — rather than on technological possibilities — businesses can rationalize and prioritize their technology offerings. As a business or technology leader, you will be able to take full advantage of the array of new technologies and the ease of accessing them. You will be able to address customer needs and succeed sooner — within a one-to-two-year time frame — at what you really need to do: transform innovation into revenue and growth.