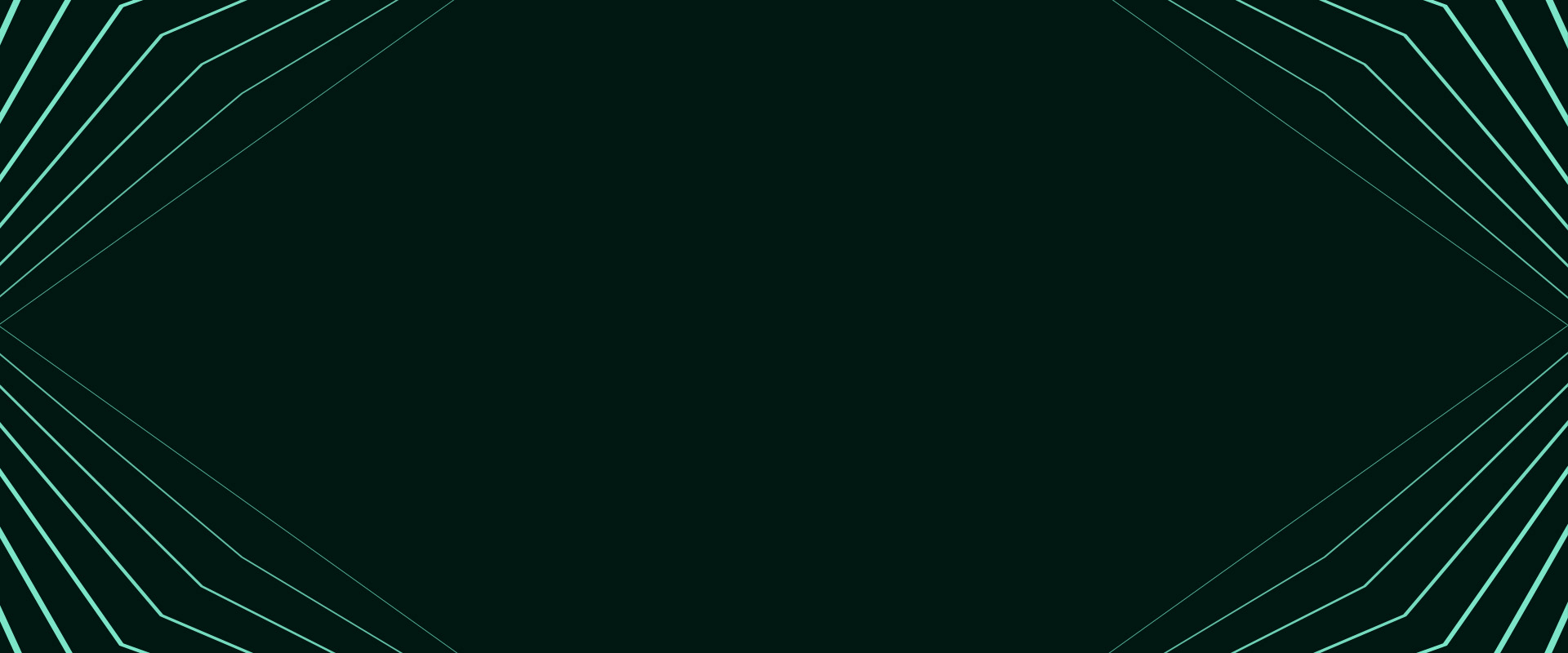
Subscribe to Insight Exchange on Apple Podcasts, Spotify, Google and Amazon Music and Audible for the latest episodes.
In this episode, we’ll explore the categories of solutions in the data and analytics (D&A) value chain and the crucial role of cloud data storage in modern D&A ecosystems. We’ll examine the different tools and processes involved in each category of the D&A value chain, from data integration and processing to analytics and visualization. Additionally, we’ll uncover the differences between legacy and modern D&A infrastructure solutions, highlighting the advantages of modern solutions such as flexibility, scalability and improved data governance. You will discover the benefits of implementing a modern D&A infrastructure, including enhanced decision-making, increased efficiency and improved customer experiences through personalized interactions. We’ll also discuss the core shifts driving the move toward modern D&A architecture and the key growth areas within the D&A value chain. We’ll shed light on the competition between Snowflake and Databricks, two leading players in the market, and explore the factors driving the market growth of both solutions. Lastly, we’ll discuss the level of competition between Snowflake and Databricks and the reasons for customer migration to Snowflake.
To provide insights on these topics, we will hear from Harsha Madannavar, Managing Director and Partner at L.E.K. Consulting’s San Francisco office; Dominic Perrett, Managing Director at L.E.K. Consulting’s San Francisco office; Jordan Barron, Managing Director and Partner at L.E.K. Consulting’s Los Angeles office; and Marco Cataluffi, Consultant at L.E.K. Consulting’s Los Angeles office.
L.E.K. Consulting is a registered trademark of L.E.K. Consulting. All other products and brands mentioned in this document are properties of their respective owners. © 2023 L.E.K. Consulting
-
Read the full transcript below
Host:
Welcome to Insight Exchange, presented by L.E.K. Consulting, a global strategy consultancy that helps business leaders seize competitive advantage and amplify growth. Insight Exchange is our forum dedicated to the free, open, and unbiased exchange of the insights and ideas that are driving business into the future. We exchange insights with the brightest minds of the day, the most daring innovators and the doers who are right now rebuilding the world around us.
Marco Cataluffi:
Welcome to today's episode in which we'll explore the categories of solutions in the data and analytics value chain, and the crucial role of cloud data storage in modern data and analytics ecosystems. We'll examine the different tools and processes involved in each category of the D&A value chain, from data integration and processing to analytics and visualization.
Additionally, we'll uncover the differences between legacy and modern D&A infrastructure solutions, highlighting the advantages of modern solutions such as flexibility, scalability and improved data governance. We will discover the benefits of implementing a modern D&A infrastructure, including enhanced decision making, increased efficiency, and improved customer experiences through personalized interactions.
We'll also discuss the core shifts driving the move towards modern D&A infrastructure and the key growth areas within the D&A value chain. We'll shed light on the competition between Snowflake and Databricks, two leading players in the market, and explore the factors driving the market growth of both solutions. Lastly, we'll discuss the level of competition between Snowflake and Databricks, and the sources of customer migration to Snowflake. To provide insights on these topics, let us welcome our experts, Harsha Madannavar, Dominic Perrett, and Jordan Barron.
Would you please each take a moment to introduce yourselves?
Harsha Madannavar:
This is Harsha speaking. I head up our technology practice globally at L.E.K. I've been with L.E.K. for about 16 years. I've also spent 10 years in product engineering prior to L.E.K., and I also head up the San Francisco office. Within technology infrastructure, we cover many, many disciplines, from semiconductors through to system and application software. Within that, data analytics ecosystem and the value chain is certainly a critical domain area alongside cybersecurity, the IT infrastructures, the industrial digital ecosystem, and getting down to compute storage, networking solutions as well, and certainly the information economy, specifically, the data sets that are derived from all of the underlying infrastructure. That's really where I spend all my time working with other partners around the globe. Half of our time is roughly transaction related and the other half directly with corporates on various growth strategy issues.
Dominic Perrett:
Yeah. Many thanks Harsha and Marco. I'm very excited to chat through today. I'm Dominic, a partner out here in San Francisco with Harsha and Jordan on the West Coast. I also sit within our data and analytics team here at L.E.K. as well as our broader tech infrastructure group. I've been with L.E.K. around 12 years or so. Yeah, I'm very much looking forward to the conversation today.
Jordan Barron:
Yes, and thank you all. Thanks, Marco and Harsha, and I'm Jordan. I'm another partner here at L.E.K. in our tech infrastructure practice rounding out the group. I've been with the firm for about 13 years and spent a significant amount of time in the data analytics value chain having worked with a lot of the venture capital firms, growth equity firms, traditional private equity firms, as well as the corporates themselves on growth strategy. We've tackled this subject from multiple angles. I'm excited to dive deeper today.
Marco Cataluffi:
Wonderful. Thank you all for the introductions. Perhaps to start out, what are the key categories of solutions in the data analytics or D&A value chain, and what do you consider the role of cloud data storage in the modern D&A ecosystem?
Dominic Perrett:
Yep. Absolutely, Marco, and maybe I'll start with this one. Before we dive in, I think it's obviously important just to emphasize the criticality of modern data architectures and their role within an organization. There's obviously a huge array of different interconnected trends that are driving investment and growth across the data and analytics ecosystem, and a genesis for a lot of this investment are the significant challenges that organizations see with traditional legacy data and analytics infrastructures. They're not very scalable. They're error-prone. It's tricky and complex to do data governance. You have many different disconnected data silos. The management and the analysis of that data is really overly centralized.
We'll spend a little bit more time later on in this podcast going through some of the benefits of a modern D&A infrastructure, but at its heart, it's really around improved data-driven decision-making driving towards better insights, fundamentally improving the customer experience, so creating more personalized touchpoints with your customers and, fundamentally, it allows you to have a more distributed self-service analytics model. You'll have data scientists and data analysts that are embedded within business units that know exactly which queries to run, and they have access to those data and analytics tools.
I think, when we break down that ecosystem, there's really a couple of critical building blocks that we define within a modern D&A ecosystem. The first is really what we call the stages of the D&A value chain. Jordan will spend some more time digging into this in more detail, but fundamentally, if you think about the flow of data from left to right, you start with all of these various data sources. Then there's a set of data management layers around that data, so everything related to data integration and processing, data middleware, so things such as data quality, data governance, data observability, the transformation of data.
We then have the third stage which we call data storage. Obviously, the role of the modern cloud data warehouse or data lake is pivotal within that data storage stage. Finally, we have everything related to analytics and visualization, which can be basic analytics, basic reporting, all the way to more advanced predictive data and analytics. That's really one core theme that we'll explore in greater detail, and then the second theme which we'll also spend some more time digging into is what we call the various D&A archetypes, especially the modern data and analytics archetypes. This goes from organizations that are doing their first tentative steps into a modern D&A architecture, so expanding beyond data silos all the way through to organizations that are really doing best-of-breed machine learning, building AI infrastructures and really getting into that next level of data science.
Marco Cataluffi:
That's really insightful, Dominic. Thank you for sharing your perspective here.
Now, moving on to the next question, as Dominic introduced the four key stages in the D&A value chain, Jordan, perhaps could you walk us through what are the key tools and processes in both, in each?
Jordan Barron:
Sure. Absolutely. I think Dominic laid out how we think about the flow of information. If we double click on that a little bit, if we think about data sources in particular, this is where you have the organization apps and other data collected and captured through various systems and tools. You can think about, say, SaaS application data. There's transaction data. It could be data captured from IoT devices or pulling in third-party data as well. That's where you have your sources of data in that data management layer where we sub-categorize that into data integration and data middleware category.
This is where you have tools that are ingesting the internal and external data that feeds to the storage solution. These could be ELT solutions like Fivetran, data flow management tools. You could have event streaming solutions like Confluent, data replication and data virtualization players like Denodo, and then, within the data middleware layer, this is where you have the process and solutions that are making data more accessible and properly managed throughout the organization. This category includes solutions such as MDM management, data quality, data government security and cataloging, installation. Then you have a bit newer categories such as data observability. You have transform within there, DPT labs as a good example, as well as we put reverse ETL in that data middleware layer such as Census.
Then we have the third category of the four is the data storage. This is the centralized home for all the internal-external data we spoke about under the data source category. These are the vendors that many have heard about and I think you introduced, Marco, as well, Snowflake, the data warehouse or data lake such as Databrick. That's where they would live, and then the last category is the data analytics and visualization layer. This is where we have basic analytics, reporting and visualization, as well as the more advanced solutions that do the predictive data analytics that are using AI and machine learning.
In the more basic category, you would have individuals such as Looker and maybe ThoughtSpot. Within the predictive analytics stage, you have vendors such as DataRobot, H2O.ai, Dataiku. Of course, you also have legacy players that span across some of these categories or all of them, and so you have companies such as TIBCO or Informatica more on the left-hand side of the value stage, and that vendors and the reporting analytics layer such as Tableau, Power BI, some of the vendors that a lot of organizations have probably been accustomed to using over the last number of years.
Marco Cataluffi:
Thank you, Jordan. That's a unique way to look at the D&A value chain. Thank you for the detailed answer.
Now, turning our attention to the next question, what do you consider to be the key differences between legacy and modern D&A infrastructure solutions?
Harsha Madannavar:
The legacy solutions have been around for a long time for obvious reasons, but they weren't designed to handle the large volume, the variety and the complexity of data and the type of use cases that are intended to be developed and served up with the type of data that we are coming across today. I think some of the constraints from legacy solutions is really about their ability to manage that vast array and depth of data to really help in downstream analysis, reporting, et cetera, whatever the specific use case might be. The second is they were designed for a user to be highly technical as opposed to a business user or a business analyst and be more self-help, self-service oriented.
Those are some of the classical constraint, not to mention the fact that they're largely on-prem deployments versus some of the more modern solutions today which are largely cloud Native, and inherently with that comes all of the benefits of being in the cloud, flexibility, scalability, et cetera. More importantly, all of the regulations and governance constraints that are being introduced by jurisdiction, et cetera, are probably easier to deploy when you're looking at a modern implementation. Being in compliance with privacy, data governance policy enterprise-wide down to specific departments, but also introducing the necessary security compliance programs in place I think is critical.
The other aspect to modern is really serving up the solutions directly to the individual and stakeholders who are consuming it, so self-help, self-service orientation, where they can run a lot of the analysis themselves directly on the source data sets. Those are much more conducive when you have modern deployments versus some of the constrained situations of legacy systems.
Marco Cataluffi:
Wonderful. Thanks, Harsha. I'm really glad that you brought up some of the benefits of implementing a D&A infrastructure. On a related note, I wanted to ask what do we consider to be the benefits of implementing a modern D&A infrastructure compared to a traditional or more legacy infrastructure?
Dominic Perrett:
Yep. Absolutely, Marco. Harsha obviously covered a few of those items at a high level as well. I think it's helpful, too, if you maybe dial the clock back five to six years as the modern D&A infrastructure was really getting off the ground. I think you were seeing a lot of investments happening on the right-hand side of that value chain that Jordan was articulating, a lot of investment happening maybe in some of the more basic or early analytics and visualization tools such as a Tableau or a Power BI.
I think what you are seeing from organizations that were making these initial tentative steps investing on that right-hand side of the value chain is they were really putting the cart before the horse with regards to the investments that they were making. They were getting to a point where they had more modern analytics tools layered on top of the more legacy data infrastructure, and they really weren't able to see the main benefits and the ROI on those investments that they were making.
Really, the benefits of a modern D&A infrastructure, if you think about everything as it relates to connecting various data sources around data management, around data storage, it really is geared around improving decision-making processes as it relates to data. Data today really is at the core and the heart of a modern organization, so you're able to analyze and query data in real time. You improve the overall accuracy and, therefore, the insights that you're able to extract from that data, and that really is one of the key critical benefits.
You also drive, frankly, a lot of efficiency and the potential for a lot of cost savings. Yes, obviously, there is an up-front investment to be made. There's a lot of services organizations that live in and around that D&A ecosystem that are able to architect and implement a truly efficient ecosystem for organizations, but over time, that drives automation and streamlining of processes.
Then there's also the tieback to the customer experience if you think about organizations, the relationships that they have with their end customer. The customer is demanding an increasingly personalized experience with that company. As the organization collects these ever larger volumes of data, they're able to leverage more sophisticated analytics tools to drive more personalized experiences, drive increases in customer satisfaction, and fundamentally improve loyalty and retention and stickiness. Obviously, tied to that are all the benefits of competitive advantage, so more agility, greater responsiveness, improved opportunities for innovation.
Then Harsha also touched upon this, and I think this is a critical piece, which is it moves the analysis and the querying of that data from a centralized IT persona into a more distributed data scientist, data analyst who is embedded within the business unit. They're obviously closer to the use cases for that data. They better understand how do I need to use my data, what insights am I trying to extract, so they're able to use their skillsets within that given business unit to really drive improved power from the data that the organization has.
Marco Cataluffi:
Thank you for the thoughtful response, Dominic.
Now, let's pivot slightly and talk about what enables organizations to capture the benefits of a modern D&A infrastructure that you just discussed. What are the core shifts driving the move towards a modern D&A architecture?
Jordan Barron:
Sure. I can take this one, Marco. If we look at the work that we've done over the last number of years, we've identified about a dozen or so fundamental shifts that map back to the categories across the D&A value chain that we talked through at the beginning of this. I'm not going to go through all of them, but I did want to highlight a few. Maybe the first one I would like to talk about is the move from ETL to ELT. ELT, or the extract, load, transform, is a modern variation on the older process of extract, transform, and load, or ETL, in which transformations take place before the data is loaded.
Running transformations before the load phase results in a more complex data replication process. Because ETL transforms data prior to the loading stage, it's really ideal when a destination requires a specific data format. However, if you're storing your data in, say, cloud native data warehouses such as Redshift or BigQuery or Snowflake or Databricks, we see that ELT as a much better approach. Your organizations can transform their raw data at any time when and is necessary for their use case and not as a step in the data pipe.
A second one to highlight and related to the shift from ETL to ELT is in the middleware stage with the shift of manual process to automated data transformation. Here, companies such as DBT allow organizations to optimize their antiquated data transformation processes and build data pipelines to make data more accessible. What this enables is the ability for organizations to track dependencies and improve data query ability.
I think another one to highlight from Harsha and Dominic would be the shift towards self-service insights. Turning analysts into citizen data scientists or business analysts enables organizations to develop customized output and insights with the data. Organizations are now armed with powerful software so they can perform more detailed diagnostic analyses, create machine learning models and supplement their work and just draw better insights from the data at hand.
I think those three are pretty key to the highlight, but there are about a dozen or so overall.
Marco Cataluffi:
Wonderful. Thank you, Jordan. That was really informative. Now let's shift gears a bit and talk about the next topic. What are some of the key growth areas in the modern D&A value chain?
Harsha Madannavar:
We are seeing growth really across the board. I think, if you look at the nature of the infrastructure or the pipeline that have been set up in various organizations, it's varying degrees of maturity. We are seeing the ability to run analysis at the right level being mixed actually. We're seeing investments in upgrading the infrastructure right from the start to the end. Think about the data sources itself, whether it's structured or unstructured data format. We're starting to hear areas around pulling data from IoT devices as an example. You're starting to collect all kinds of telemetry from different sources of information, whether it's the application, whether it's the device, the individual behaviors, et cetera.
The source of the data itself is one where we are seeing product design being developed to allow for more reading of information, number one. Number two is data integration. Now, data integration has been around ever since the data infrastructure of 30, 40 years itself, but the ability to integrate different types of data feeds in whatever frequency is needed, that's an area of investments, whether it's IPAs, whether it's CDC, whether it's proprietary connectors between applications, et cetera. Even within integration, there's different types. Whether you copy the data, move the data or just transfer just the metadata itself has implications around consumption of compute security doing it in a compliant manner. There's different techniques in which data can be integrated itself. The flexibility of allowing for integration at scale and on the demand is important.
Certainly, there's a lot to be said around the analysis itself, and there's a lot of innovation going on in the analytics engines, whether it's supervised, unsupervised machine learning, and certainly with all of the themes that are going around with generative AI. I think that just introduces another demand in terms of innovation on that layer, so the ability to bring together data sets in order to train large language models in a compliant and safe manner so you've got the quarantine around the data sets. Those are areas that we're seeing a lot of innovation. Perhaps, it'll lead to the revival of on-prem infrastructure in a very large way as an example.
We're seeing investments in data pipeline specifically around moving data between source and target destination. Certainly, a lot has been set around visualization already, and I think that will continue to be an area of development, but more importantly, it's utility for an individual stakeholder that wants to consume it in a certain way.
One area that we're starting to see very, very early stage investments is particularly around the area of observability, just to be able to have an audit trail on data as it progresses from source to destination, and checking the reliability of the analysis because you're able to verify the authenticity and the relevance of specific data feeds.
Those are just some examples, but, frankly, we're seeing investments across the board because every organization can sit in different levels of maturity in terms of its own data infrastructure.
Marco Cataluffi:
Those are fantastic points, Harsha. Thank you.
Now, if we take a step back, we discussed differences between modern and legacy infrastructures, discussed the benefits of implementing in growth areas of a modern tech infrastructure. What is the current state of the market share between legacy vendors and modern solutions in the D&A industry?
Jordan Barron:
Sure, Marco. We've been tracking and sizing this market for a number of years. When we look at the historical market sizes and growth rates, what we've seen is that the legacy vendors have, of course, taken a greater share of the market spend, but modern solutions have been growing I'd say on total up to twice as fast overall and, depending on the category multiples, as fast as legacy solutions such as the data integration and processing stage. What we're seeing is that moderate solutions now enable expanded use cases across business units and introduce additional decision makers. As these business unit leaders push for expanded use cases, additional budgets are being allocated to spend on these solutions, but what we're seeing is this share shift almost being about 50/50 today.
There's continued innovation across the data analytics value chain both from established modern players as well as from more emerging or nascent providers in particular. I think there are certain categories in particular that we're seeing having outsized investments such as the middleware layer. You have companies within data observability, reverse ETL getting hundreds of millions or billions of dollars of investments over the last number of years. We continue to see a significant shift towards modern solutions on which will become the dominant form of the market spend over the next number of years.
Marco Cataluffi:
Thank you for the thoughtful response, Jordan.
Dominic, earlier, you mentioned how organizations can fit into different archetypes based on what tools they deploy, but what are the four distinct archetypes of the shift towards a modern D&A infrastructure, and what are the characteristics of each stage?
Dominic Perrett:
Yep. Absolutely, Marco. I think, yeah, obviously, as we walk through the archetypes here, it's important to keep in mind that the concept of a modern data and analytics architecture is by no means monolithic. There's obviously many different flavors and types of D&A architecture that an organization can deploy, and we've really identified four predominant archetypes among the organizations that we've seen that have already started and are well-advanced in their journeys towards more of a modern approach.
The first step is what we have termed the centralization stage, and this is really where organizations are expanding beyond the data silos that may characterize more of a legacy architecture. Typically, what you see here is the initial adoption of a cloud data warehouse and the associated data integration and some data processing needs in order to support that migration to modern data storage. Again, this has huge variation across the different organizations, but typically this might look like a migration to a Snowflake or a Databricks. Maybe an organization is using one of the hyperscalers, so Amazon, Azure, and then they're utilizing various data pipes, be it an ELT, ETL process, to move the data from existing silos into that centralized storage location, so maybe it looks like a Fivetran connected with a DPT and Snowflake is one relatively common combination of tools.
If we think about the organizations that have actually already begun their journey towards a modern data architecture, we actually still see the majority of those organizations within this first archetype. Even though transition to modern data architecture is still in a relatively early stage, but once you've begun that journey, there's still relatively few organizations that have moved beyond that first archetype.
The second archetype is what we call the visualization and optimization stage. This is where you start to really drive the core benefits of a modern architecture. Maybe you're implementing real-time use cases. There's a lot more focus on data governance and data cataloging and security. This is where we see tools such as Collibra and Alation initially deployed. It's also where those investments that are happening further down in the value chain really start to bear fruit, so getting into your reporting and data visualization use case, and so maybe utilizing tools such as Looker or ThoughtSpot on top of a modern data warehouse to really drive the benefits of having data within a centralized location.
The third step which we term the intelligent product stage is starting to move beyond structured data into either unstructured and semi-structured data and really starting to utilize data lakes which, obviously, more typically house unstructured semi-structured data relative to a data warehouse. You may see tools such as event stream processing being used to a greater extent, so Confluent as an example. Maybe organizations will start to deploy Databricks alongside Snowflake in this particular architecture.
You're also starting to see more of a preliminary experimental usage of more predictive data analytics, and that really then feeds into the fourth stage which we call the D&A excellent stage which is where you're really utilizing best-of-breed machine learning and AI to its greatest extent. This would be organizations where you have truly data scientists and data analysts embedded in every single business unit. You may even have a centralized machine learning-oriented team that is focused on driving the best possible tools. DataRobot, Dataiku, as an example, would be some of the tools that you're starting to see in this fourth and final stage.
As mentioned, if we take those organizations that have begun this modern journey, they're still very much clustered around that first archetype. We do see organizations that are making the initial steps into the second stage and the third stage, and then it's really a select group of leaders that are even touching the D&A excellent stage.
Marco Cataluffi:
Thank you, Dominic. This is very fascinating, and it's clear that a lot of thought has gone into this research.
Now, as we continue our conversation, I'd be interested to hear some thoughts on what do you consider to be the key drivers for organizations to transition to a more sophisticated D&A infrastructure?
Harsha Madannavar:
It hasn't really changed. I think it's fundamentally around what we would expect, which is driving automation and then the quality of decisions that is being done today in terms of improving the efficacy of decisions through better data. Now, that might seem trivial. It's obviously a question that has been around since the dawn of IT infrastructure. The fundamental questions haven't changed, but I think the pursuit of some of those capabilities is certainly reaching a higher threshold because of the ability to extract and utilize data from different streams to inform the scope of automation and the scope and efficacy around decision support.
I would say the key driver is, number one, organizations realizing that they can take advantage of some of the data sets that they're getting access to to achieve some of those outcomes that they're looking for. It's a broader recognition that there is something they can do to get more, which will drive the investments in a modern data infrastructure that might be appropriate for what they're trying to achieve.
I'd say, in that, there's probably an understanding that they may not have the foundations to serve a broader digital transformation or modernization effort, and so investments to modernize the underlying architecture to make it conducive to serve up different applications and so on and so forth. Certainly, not everything is being conceptualized today, but investments to lay the foundation is a key driver there, and that is either recognized at an organizational level so it's serving all divisions, all stakeholder types or it could be at a functional level as well. Those are some of the examples there.
I'd say, as you get more sophisticated in the utilization of your infrastructure, you start to think about other issues. How can you optimize the resources that you're utilizing, whether is compute, storage, et cetera, your cloud workloads as an example? How can you reduce the frequency of error, increase the reliability and the data quality that's being served up.
Implementing the governance processes at scale and ensuring that there is no redundancy around data types and so no one's fundamentally questioning the underlying fact base that there is a, quote, unquote, golden source of information that is appropriate to serve up in combination with other data sets, whether it's the master data, with transactional data or reference data as an example, so really making sure that there is a proper view on how an organization's data assets are being set up for maximum benefit, I think that is really a big driver that underpins a lot of the investments.
Marco Cataluffi:
Thank you for the detailed response, Harsha.
If we take a step back, we discussed what the different archetypes are, what drives organizations to deploy a more sophisticated D&A infrastructure. What percent of organizations have truly started their modern data infrastructure journey, and how many have moved beyond the second archetype?
Jordan Barron:
Yeah. We're still in the early innings of this journey to a fully modern suite of solutions. We do see a significant white space across the market. If you look at the data, we're seeing that about 30% of organizations have truly started their modern data infrastructure journey, which means about 70% are still using legacy D&A infrastructure. If we think about the 30% that are using modern D&A infrastructure, we're estimating about 16% of these organizations have moved beyond archetype two. We see a significant amount of near-term addressability amongst the legacy vendors. We see about two-thirds of those are near-term addressable, and maybe another one-third are long-term addressable, but we see all of that legacy infrastructure addressable for the modern D&A vendors.
There's a lot of organizations looking to make their initial move out of legacy solutions. I think the businesses, if you think about the investment, time of deployment, speed to realizing ROI, it just has improved significantly over the past few years, and it's expected to be the case moving forward, so we see this propelling investment and wider adoption of modern D&A infrastructure going forward.
Marco Cataluffi:
Great. Thank you. Thank you, Jordan.
To close the conversation, we would like to thank our expert guests for the discussion regarding data and analytics ecosystem, software and services, including market perspectives and case examples. We're happy to provide more detailed discussions on requests, and we invite you to connect with us to learn more about how L.E.K. Consulting has extensive experience in providing strategic support to subscription-based and growth-focused businesses and investors who must focus on data and analytics such as data integration and processing, the benefits of implementing a modern D&A infrastructure, and improving efficiency, as well as customer experiences through personalized interactions.
Host:
Thank you, our listeners, for joining us today at the Insight Exchange, presented by L.E.K. Consulting. Links to resources mentioned in this podcast can be found in the show notes. Please subscribe or follow for future episodes wherever you listen to your podcasts. Also, we encourage you to submit your suggestions for future insights online at lek.com.
Related Practice
03012024130308