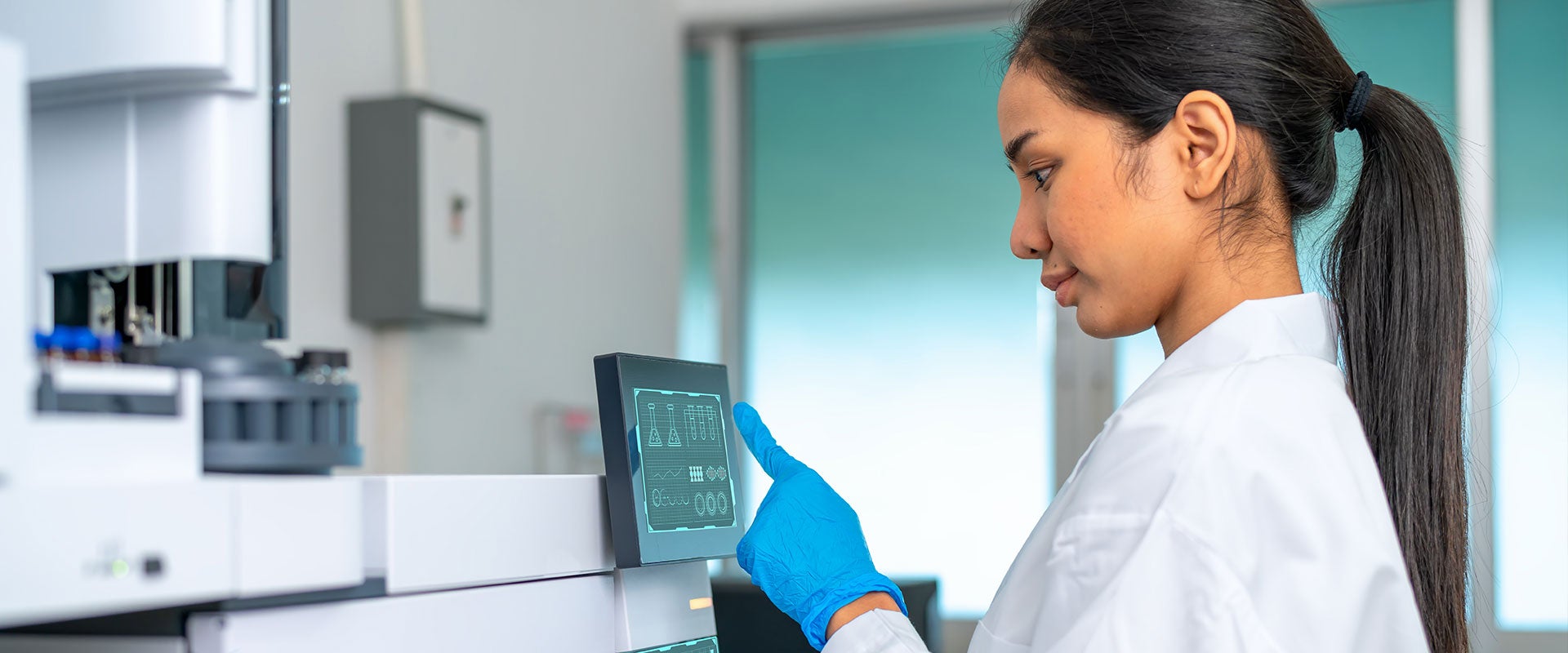
Digital Transformation of the Laboratory
- Article
L.E.K. Consulting and Everlife gathered 100 industry leaders, including manufacturers, distributors, lab operators, and investors from the Clinical Diagnostics industry, for a seminar entitled, “Diagnostics 2025 and Beyond in Asia". The purpose of this seminar was to share perspectives on the outlook of the clinical diagnostics industry over a five-year horizon — across product, technology, and testing — and how can relevant stakeholders be prepared for changes in the coming years.
This article captures the key insights from one of the sessions on the “Digital Transformation of the Laboratory”.
For societies with rapidly aging populations and an increased focus on value-based care, prevention and wellness, we must ensure a transformation of the lab to being more automated, data-first and efficient. There is a key expectation that labs will become data-driven entities in the healthcare ecosystem, acting as specialized advisors to providers involved in healthcare delivery. In this enhanced role, it is ever more critical for labs to be interconnected with the healthcare ecosystem striving to deliver solutions within a short turnaround time.
While diagnostics have increasingly taken a central position in the therapeutic paradigm, key challenges in the lab continue to be a bottleneck in leveraging the power of high-throughput diagnostics. Most critically, turnaround time and diagnostic accuracy are still hampered by process inefficiencies in the current lab setup. It is believed that up to 70% of lab workers’ time is wasted performing administrative tasks, doing preparation work, cleaning data and reporting.
Many labs continue to operate without significant automation, a key gap considering the rising cost of attracting and retaining healthcare talent in the APAC. Additionally, the capture, retrieval and reporting of analyses in labs continue to occur in a fragmented process. Lastly, a continuous overhead of administrative tasks and inventory management continues to increase inefficiencies in the lab. As one panelist highlighted, a critical requirement for labs today is effective software tools to manage both the procurement and the management of reagents for different diagnostic tests.
It is notable that key players in the field are trying to move toward integrated IT systems and data interoperability during ongoing geopolitical issues regarding data privacy and data security. A critical role for regulators across markets could be to ensure clarity and adherence to compliance guidelines that ensure safe and equitable data sharing (local and cross-border data sharing).
A transformation to this end would also enable further collation between publicly available biological data and private data to further streamline drug discovery efforts. In this context, one panelist highlighted the need for a continued commitment to enhance the privacy and security of clinical data to protect patients’ interests.
Specifically, panelists noted the key need for labs to transform from being simple data processing centers to being centers for collection, analysis, reporting and sharing of clinical big data. The increased digitalization and automation of lab processes will be a key lever in achieving this objective. Digitalization of lab workflows — starting with sample collection and preparation (e.g., use of sample barcoding, automated collection and ferrying), sample processing, result aggregation (e.g., transfer to cloud-based ELN/LIMS), and reporting — will be a key driver of the proposed lab transformation.
Additionally, panelists recommended stronger efforts to integrate with the broader healthcare system, enabling 24/7 operational times and short turnaround times, as well as the creation of data infrastructures to enable rapid access to patient data for therapeutic decision-making. In this context, the establishment of reliable infrastructure with servicing mechanisms to ensure minimal downtime was highlighted as an important requirement.
The panel concluded that digital workflows in the lab, relying on process automation, support of data analysis through AI and predictive maintenance, will be key drivers of efficiency and the reduction of diagnostic errors.
The growing role of diagnostics in healthcare delivery presents an imminent opportunity for the use of artificial intelligence (AI), expanding the role of labs from being data processors to being specialized advisors. In this context, the panel highlighted the tremendous potential of clinical decision support systems (CDSSs) in diagnostic labs and therapeutic settings. Additionally, the effective use of existing data can add immediate value to the healthcare business.
For instance, BioFire Syndromic Trends is a software feature from BioFire that provides local and regional pathogen circulation trends on demand. Users can view pathogen trends for their own lab as well as regional and national pathogen trends, all of which are created from de-identified data that has been aggregated with other participating institutions’ test data. More importantly, understanding macro trends can be crucial for strategy of and planning by diagnostic businesses.
Despite initial concerns about their accuracy, CDSSs are here to stay. A key challenge for CDSSs in the past was the high rate of errors arising due to gaps in data availability. With increasing digitalization and infrastructure for data aggregation, CDSS usage will continue to increase in the long term.
While there is tremendous excitement about AI, many applications continue to be in experimental pilots at the current time, with scalable applications for routine clinical use yet to be implemented. In this regard, the panel noted that the increased aggregation of data in standardized, usable forms would be critical in leveraging AI applications.
Additionally, concerted efforts in digitalization will be critical to leveraging these applications. For example, digitalizing 2D and 3D tissue samples can enable use of intelligent image recognition systems for rapid preliminary diagnoses and prognoses. Overall, a data-first strategy will be the key to implementing AI applications in a scalable manner to support lab technologists and physicians in therapeutic settings.
To learn more, please download our analysis.