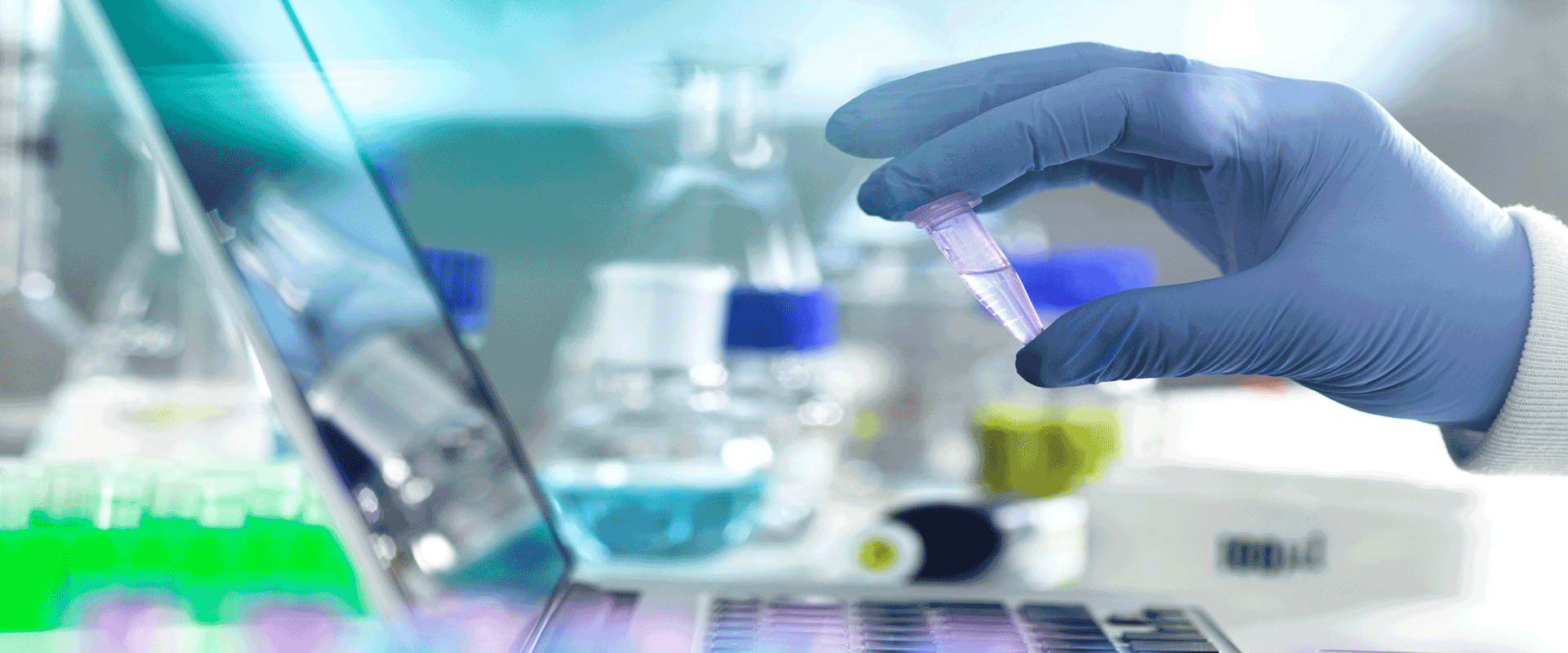
AI in Life Sciences: Emerging Business Models
- Volume XXII, Issue 28
- Executive Insights
The presence of artificial intelligence (AI) in life sciences is growing quickly as a result of multiple AI companies focusing on drug discovery and development. The momentum is set to continue — we believe that AI is at a formative stage and adoption has the potential to accelerate rapidly.
In this context, AI companies are experimenting with business model paradigms ranging from service provision to internal development of drug candidates. In this Executive Insights, we characterize the business models being adopted by AI players and their required capabilities, and provide our perspectives on the potential trajectories of AI companies — and their investors — as these companies continue to mature.
AI companies may, in theory, adopt one of two pure-play paradigms: They can either act as service providers to pharma companies (akin to contract research organizations (CROs)) or they can focus on internal development (akin to biotechs).
In the service provision paradigm, companies sell AI services to pharmaceutical clients in a model similar to that of CROs. They tend to work off clients’ datasets and success is determined by the ability to deliver the promised AI service (e.g., identifying drug targets or selecting lead candidates). In this paradigm, AI companies seek to build revenue via client acquisition.
In the internal development paradigm, AI companies act similarly to traditional biotechs by relying on their AI-powered platforms to create their own pipelines and advance novel or repurposed candidates through development. The ultimate AI-powered biotech could seek to leverage AI from target identification to clinical trial design. In this paradigm, companies aim to significantly cut R&D timelines and cost before partnering or selling the asset to big pharma, as traditional biotechs do.
Our review of the most prominent AI companies suggests that, in the majority of cases, companies initially adopt a “mixed model” including elements of both service provision and internal development as they seek to develop, test and validate their AI-powered platforms (see Figure 1).
On the service provision side, some AI companies start by primarily collaborating with academia or charities. The aim, beyond the notoriety of the relationship, is to test and improve the AI through access to data or knowledge. These relationships are typically not financially oriented so they are easier to form and manage than are those with pharma. AI companies then look to expand to pharma clients. This is most commonly on a payment-by-results basis, which de-risks the investment for the pharma client and may help overcome skepticism. In parallel, AI companies also tend to develop their own pipelines, which in the AI world does not require the extremely high levels of funding seen in traditional biotech.
Although combining service provision and internal development may initially seem surprising, the approaches are complementary and self-reinforcing for an AI company as it develops (see Figure 2).
Working on internal programs is a powerful way to convince both investors and potential customers of the value of the AI platform. Successes can be publicized and use cases demonstrated to attract customers to the service side. This helps overcome the fear that the benefits of AI may not be sufficiently tangible, which is often cited as a key barrier to AI adoption by biopharma. Offering services in parallel also builds credibility, as the quality and size of the customer base is often seen as a key performance indicator by investors and potential customers. Service provision also generates funds that can be deployed to finance internal programs.
We found few exceptions to the mixed model. Pure AI-powered biotechs tended to be companies very well funded from the start (e.g., BERG) or that use an extremely light R&D process (e.g., in repurposed drugs).
AI companies are growing and agile and can experiment with both business model paradigms. As companies mature and develop, we think that the different skill sets required will mean they will move toward specializing as service providers or AI-powered biotechs to remain competitive (see Figure 3). Over time, investors are likely to specialize too — only a few private investors will want simultaneous access to both a service business and a scientifically risky, biotech-like venture.
The market-leading AI-powered CROs of the future will need to display deep AI expertise, remain at the forefront of innovation and likely maintain a differentiated offering. They will also need sophisticated business development approaches targeting key R&D stakeholders in potential pharma customers, as well as the ability to embrace a customer-oriented approach.
In contrast, emerging AI-powered biotechs will need to have internal teams that are able to pick attractive areas of focus from scientific, clinical and commercial angles. Pharma-facing efforts will target a different set of stakeholders, most likely business development teams for potential late-stage clinical partnerships. These companies will also require broader understanding and capabilities across the R&D spectrum as pharmas take responsibility or outsource to CROs for a broader set of stages. AI-powered biotechs may not need to remain at the cutting edge of AI, as long as they are able to efficiently deliver drug candidates with sufficient commercial potential. This is because they will compete for funding and ultimately revenue against “traditional” biotechs to bring drugs to market rather than compete for the business of pharma clients with other AI companies.
As the market moves to maturity, succeeding in both service provision and internal development will likely prove challenging, except for larger entities with access to significant resources. Over time, we expect both models to prosper and to see more companies choosing to specialize in the area where they have the greatest competitive advantage.