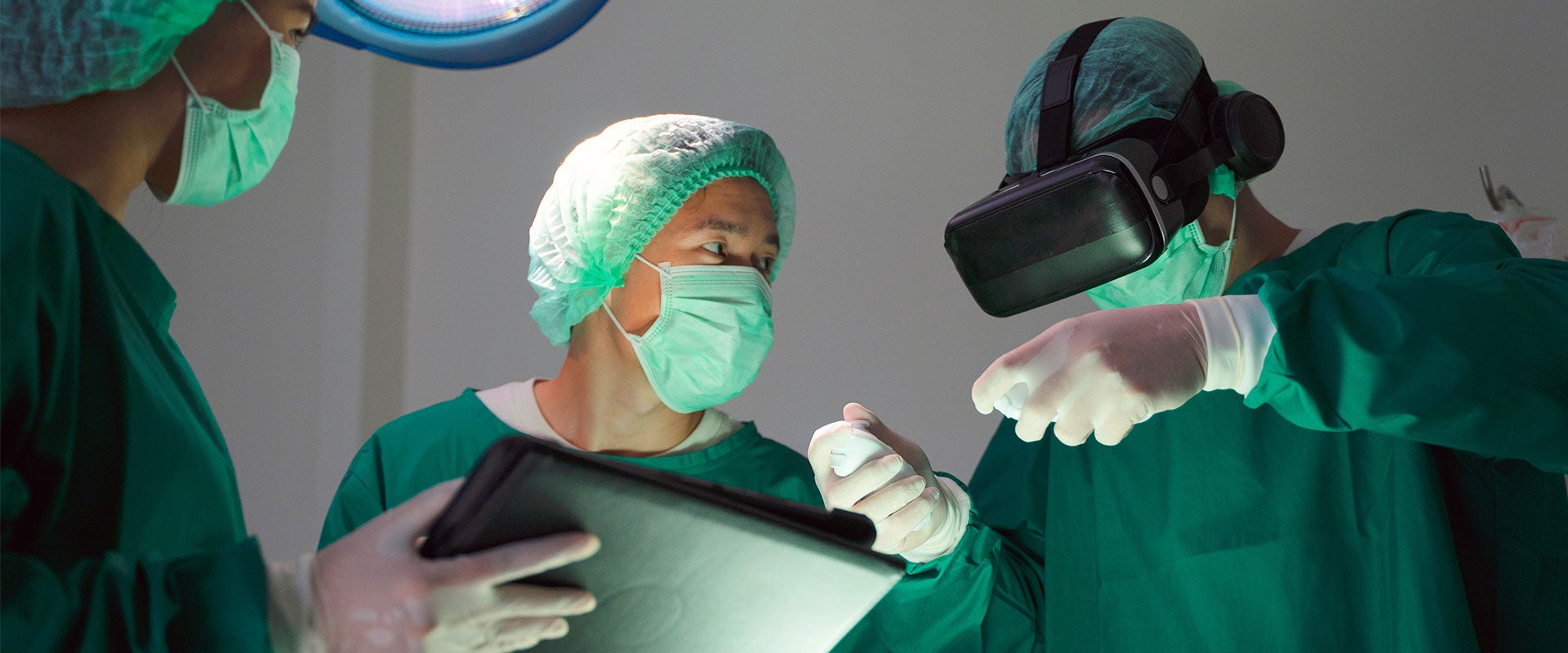
Editor’s Note: This report originally ran on the Medtech Strategist website on May 30, 2023
Artificial intelligence (AI) will disrupt how medtech companies do business, whether or not they choose to embrace it. Organizations can either act and invest now or be left scrambling when time and resources are scant and the competition is well ahead.
AI is fundamentally a tool; there is a multitude of applications for it, and much of the challenge for your organization is to figure out to which specific use cases AI is most applicable in your organization. There are both internal applications (e.g., using AI to improve internal processes) and external applications (e.g., improving end products and services for customers). Externally, AI holds promise for further enabling the “quadruple aim” by improving patient outcomes, improving provider efficiency and costs, and improving the experience for both patient and clinician.
AI has transformative potential, and mobilizing your organization to identify high-impact opportunities, trial a few AI applications and build an AI-centric culture to guide the organization will be paramount to your firm’s success against the competition. AI applications appear endless, but to help you get started, a few key use cases for medtechs include operations, sales and marketing, and importantly, product- and service-related applications. Your firm’s first AI application may not be the most impactful one, but it will help your organization begin to build its capabilities and team for future applications.
External applications
Product- and service-related applications
AI has tremendous potential given the vast amount of untapped biometric data. This category may be the hardest to accept fully, which is why the next section outlines it in more detail. Broadly, AI is going to change healthcare in areas like real-time patient triage, image-enabled diagnostics, and cardiac and neural electroceutical therapy (i.e., cardiac rhythm management, neuromodulation). Some forms of AI will be the end product/service, while other forms will be part of the total service (e.g., services surrounding the algorithms).
If your product/device currently generates data or could one day generate data, AI should be an integral part of your strategy going forward. Devices leveraging AI may be better able to illustrate effectiveness, such as a heart monitor that more effectively detects atrial fibrillation (AF), a continuous glucose monitor (CGM) that predicts a likely patient crash based on a variety of inputs, or even an orthopedic implant that predicts and plots patient rehab recovery following a knee replacement based on physical therapy progress to course correct patient and healthcare provider (HCP) involvement more quickly.
From a service perspective for patients, AI-powered patient monitoring may substantially reduce emergency room visits (or optimize them when they occur), enhance the provision of real-time health information such as urgent care coordination (e.g., Viz.ai stroke care coordination) and improve chronic disease management.
HCP shortage
As we ask doctors and nurses to cover more patients despite there being fewer HCPs, the historical hesitancy to adopt new technologies will give way to a generation of HCPs who grew up with smartphones and the internet. Insertable electronic devices (e.g., pacemakers, implantable cardiac monitors (ICMs)) are an excellent example of the use of intuitive dashboards through which vast amounts of data are being analyzed in real time and a single HCP can view their entire patient panel from a single station. As the U.S. population continues to skew older and sicker, HCPs will become increasingly reliant on — or perhaps empowered by — AI as a critical tool.
Internal applications
There are several internal applications for AI (see Figure 1).
Operations (e.g., Olive, Resilinc, e2open, MUUTA)
AI is well suited to operations, where processes generate vast amounts of data and repeatable, rule-based decisions or tasks occur. Examples are robotic process automation, process mining, manufacturing predictive maintenance and analytics, inventory and supply chain optimization, and even invoicing (see Medtech Strategist’s Generative Artificial Intelligence: Who (or What) Wrote This?).
Customer service
AI can be a front-line customer service tool, providing customers a better experience for faster and more fulfilling answers to their questions while also providing the classic backstop/bailout for customers who prefer to “press 0 to speak to a customer service representative.”
Sales and marketing
AI is curiously becoming increasingly relevant in sales and marketing. The potential includes sales examples such as sales forecasting, lead generation, chatbots, AI-based sales coaching for sales employees, sales analytics, faster sales quoting, expedited bidding cycles for hospital purchasing groups and even sales compensation incentives. Marketing has in some ways also been a proven testing ground for AI, and leveraging AI in marketing analytics, personalized marketing and context-aware marketing for medtech customers will be crucial to bringing medtechs closer to their customers in the future.
What is driving the external AI renaissance?
To better understand what is driving the use of AI in the medtech ecosystem, we can deconstruct and evaluate several independent dimensions. The emerging confluence of these dimensions is changing the way you and your competitors are viewing the quickly approaching future of AI in medtech.
Data acquisition driven by prevalence of sensors (including wearables): The increase in the use of sensors, from implantables (e.g., pacemakers from Medtronic and Boston Scientific, smart knee implants from Zimmer Biomet) to patch-based technologies (e.g., CGMs from Dexcom and Abbott, ambulatory electrocardiograms (AECGs) from Zio and Baxter/BardyDx), and the growing presence of wearables (e.g., Apple Watch, the Oura ring, smart clothing) are exponentially increasing the data available for AI to analyze.
Connectivity: This applies to both device data export (e.g., cellular, Wi-Fi, Bluetooth) and device-to-device (e.g., med device to smartphone or med device to med device such as a CGM-insulin delivery combination). The connected environment we live in means patients no longer have to visit a doctor for a follow-up visit to analyze data (e.g., from a traditional Holter monitor) and can instead transmit data wirelessly and do it in an affordable, comfortable and convenient way. Further, it means devices can now communicate with each other, with smartphones as the hub, as they combine consumer friendliness with a powerful processor and are free for the medtech original equipment manufacturer to leverage.
Interoperability between systems and corresponding stakeholders: Healthcare is increasingly consumer driven, as patients are demanding that approach to medicine. For example, the ability to share data from the consumer to the primary care physician to the cardiologist (e.g., Philips/BioTelemetry/CardiologsAI and Apple Watch ECG data) or vice versa (e.g., Epic MyChart) will continue to improve with the passage of the Office of the National Coordinator for Health Information Technology’s Cures Act Final Rule.
Combinations of data sets: The above drivers yield the potential for previously disparate data sets to be combined. Further, they can do so for a point in time or longitudinally. Take, for example, the ability to track a diabetic patient’s disease progression by cross-analyzing CGM data, insulin data and other diagnostics (e.g., echocardiograms, urinalysis, eye exams) to better understand and predict diabetes complications such as retinopathy, foot ulcers, kidney disease and heart failure. We are only scratching the surface of what AI can do with siloed data sets; the generation of cross-device data sets, both at the personal/individual level and at the population health level, may prove transformative.
Reimbursement environment: The reimbursement landscape for AI is rapidly changing, but there is still a long way to go in figuring out how to properly reimburse for value created (i.e., value-based payments with AI-driven software are understandably more complicated than a device-based fee-for-service world). One common reimbursement mechanism is the new technology add-on payment (NTAP) (rather than physician reimbursement via Current Procedural Terminology (CPT) codes or hospital reimbursement via Diagnosis Related Group (DRG) codes), which provides an incentive for hospitals to use new and more expensive technologies not covered under a DRG during the initial years of adoption through Medicare. NTAP benefits are available for the products’ “newness” period, typically two to three years from Food and Drug Administration (FDA) approval/clearance, which foreshadows reimbursement changes for AI on the horizon.
Certain AI-based firms (e.g., Viz.ai, Aidoc, Rapid.ai, Avicenna.ai, Caption Health) have received NTAP approval in the past few years. Other firms, like Digital Diagnostics, which eventually gained CPT coverage for its IDx-DR technology, show the potential of AI in improving care. The Centers for Medicare & Medicaid Services recognized AI’s clinical utility and awarded it a higher rate than historical clinician-based reimbursement rates. Again, AI has a long way to go but there are some success stories emerging already.
The combination of the above drivers results in more data, and AI is a powerful tool when used for sorting through vast amounts of data. AI can sift through years of data and distill it into the critical details an HCP can use to optimize patient therapy. AI will never replace clinicians, but it can empower them to see critical data points that would otherwise be obfuscated by data overflow (see Figure 2).
Areas to watch
According to the FDA, radiology and cardiology are the most common therapeutic areas (TAs) where AI- and machine learning (ML)-enabled medical devices are marketed today, and they have shown explosive growth in the past decade (see Figure 3). We created a list of categories we think are interesting and will be facing transformative disruption driven by AI in the coming years. Regardless of the TA, AI will be imperative to all medtechs that are developing personalized medical devices for the future.
‘Smart’ implants and external patch-based devices
-
Cardiology: Cardiovascular conditions continue to be a top driver of mortality and healthcare costs in the U.S., and luckily, cardiology is ripe for data acquisition and analysis (e.g., pacemakers, implantable cardiac defibrillators, cardiac resynchronization therapy, AECGs, ICMs, and cardiac mapping and ablation). In September 2022, The Lancet published a paper on the role of AI-guided screening for AF leveraging an AECG, which illustrated an increased yield for AF detection and a promise for improving AF screening. The amount of electrical, pressure/flow and other biomarker data generated over a lifetime can be overwhelming, and that’s where AI is well suited to tracking chronic disease progression and flagging meaningful changes over time (e.g., following screenings of AF patients, tracking longitudinal data on the impact of lifestyle changes, medication management, interventional therapies and pacemaker implants).
-
Diabetes (and insulin delivery): Those affected by diabetes is another enormous population (37 million in the U.S. with either type 1, type 2 or prediabetes) that AI can address, and the data from CGMs, blood glucose monitors, insulin delivery pumps and patches also represents a wealth of data for AI to sift through. A recent study presented at the 82nd Scientific Sessions of the American Diabetes Association in June highlighted by Twin Health’s AI-powered Whole Body Digital Twin technology illustrated the highest reported rate of remission (i.e., as sustaining normal blood glucose levels for at least three months without taking diabetes medication) of type 2 diabetes to date. Twin Health’s twin precision treatment technology (personalized and integrated nutrition, sleep and activity guidance) was compared to standard care on changes in A1C.
-
Neurology: Neuromodulation (e.g., for pain, urinary issues, respiratory conditions, epilepsy), and more broadly mental health, are similarly data-rich TAs where AI is likely to play a transformative role, though it may be a longer timeline and perhaps less likely to come to fruition at scale due to challenges in data acquisition (or completeness of data) and complexity of the brain relative to the more straightforward TAs discussed above (relatively speaking). That said, this could be an immensely powerful use of AI to diagnose earlier and even treat the most complicated of diseases (e.g., Alzheimer’s, depression).
-
Imaging and diagnostics: A variety of siloed data sets may, when integrated, revolutionize healthcare. Many of the first use cases within any given TA are driven by radiology. AI can be a force multiplier, quickly sifting through vast amounts of data and identifying sample outliers (e.g., faint spots on images, unusual parts of an image that were not the focal point of a particular human review) that may turn reviewing clinicians into “super” clinicians who can review 10 times more images or perform a deeper review of flagged outliers where closer human review is warranted. Image-based analysis, being one of the busier areas, has a variety of applications, from improved diagnostics to procedural optimization and from chronic disease management to critical workflow decisions (e.g., for strokes). Similarly, vital signs from wearables as well as broader multiparameters (e.g., combining imaging, vital signs and samples) can have profound potential for chronic disease management, diagnostics and critical workflow decisions. Examples of imaging and diagnostics tools include:
- Imaging (e.g., X-ray, MRI, ultrasound, fundus imaging of the eye, thermogram)
- Vital signs (e.g., body temperature, pulse rate, respiration rate, blood pressure) from both medical wearables (e.g., AECGs) and consumer wearables (e.g., Apple Watch)
- Samples (e.g., blood, urine, stool, saliva, nasal swab)
-
Lab equipment machine uptime/reliability (e.g., analyzing and predicting product failures to avoid costly customer downtime)
-
Surgical tool/clinician movements: Human variability can affect every procedure, and robot-assisted surgery may provide a natural conduit for measuring and tracking techniques over thousands of procedures to identify in a quantifiable manner what makes certain surgeries more successful. AI could leverage clinician- and/or patient-specific data to optimize procedures (e.g., workflow, timing, clinical approach).
How can medtechs prepare for a future with AI?
So, where do we go from here? AI is going to play a pivotal role in the future of healthcare. Its applications are growing in both number and impact. We posit that it could create an organizational culture of embracing AI disruption within a firm, and actively investing in AI-related projects will be a key differentiator for the future winners in medtech. Curating AI for the specific use case(s) in your organization will take a combination of internal commitment, external expertise and inspiration from competitors in healthcare and disruptors outside healthcare. Dissecting where AI can make an impact in your organization will take time, and further, implementing AI and allowing AI efforts to come to fruition will take long-term commitment and fine-tuning (see Figure 4).
Given the rapid pace of change driven by AI, we recommend the following:
-
Assign ownership to determine your AI strategy. While AI is likely to affect multiple functions within your organization, there needs to be an initial owner to help create internal knowledge and deploy AI-based projects. A 2019 Harvard Business Review article details a hub-and-spoke model that may be key to your firm’s bringing in or assigning expertise but also allows for the skill set to be deployed throughout the firm.
-
Set goals and identify opportunities. Determine what goals are reasonable for the near term (2023), the midterm (2024-27) and the long term (2028+).
- Who will be your organization’s AI champions?
- Where can AI impact your organization?
- Where can AI impact your customers?
- What are your competitors doing with AI?
- What disruptive companies may become your competitors?
- Who are your potential AI partners?
- What is your monetization strategy? What could future alternative payment models (e.g., risk sharing, gain sharing) look like?
-
What is a realistic time frame for payoff? (It may not be immediate.)
-
Allocate resources. Set a budget dedicated to accomplishing your goals.
-
Move quickly; move today. AI is scary — there is a lot of technical detail. This is not your basic math that every executive knows well. This is the cutting-edge future and the innovation that will drive growth for the next decade.
-
Think about/treat your AI efforts like a tech startup would. Fail fast. Learn. Evolve. Obviously, there are critical clinical and regulatory requisites for any commercialized product to be a well-established program. That should not scare away organizations from iterating internally on early attempts at getting into AI; the process can and should breathe some tech inspiration into the organization.
A sincere thanks to the colleagues who helped in various parts of the research and editing phases of this article — Tom Bodziony, Eli Berlin, Momoka Kitara, Boyd Housey, Jay Patel and Yoni Krupski — among countless others who have been involved in a variety of projects and conversations along the way. Thanks also go to:
- Ton Luk, Manager (San Francisco)
- Ilya Trakhtenberg, Partner and Managing Director (Chicago)
- Monish Rajpal, Partner and Managing Director (New York)
- Jonas Funk, Partner and Managing Director (Chicago)
Related reading online:
- Mystrategist.com, “Caption Health’s CE Mark Story: No Walk in the Park for AI Medtech.”
- Mystrategist.com, “Caption Health: Transforming Cardiac Imaging with AI.”
- Mystrategist.com, “AI Software Nets a Medicare Win, But CMS Remains Cautious in the Space.”
- Mystrategist.com, “FDA’s AI Evolution: Perspectives from an Innovator and a Regulator.”
- Mystrategist.com, “RapidAI: Toward a Timeless Assessment of Stroke.”
- Mystrategist.com, “Digital Diagnostics’ Autonomous AI Technology Clearing Reimbursement and Payor Hurdles.”
For more information, please contact healthcare@lekinsights.com.
L.E.K. Consulting is a registered trademark of L.E.K. Consulting LLC. All other products and brands mentioned in this document are properties of their respective owners. © 2023 L.E.K. Consulting LLC
08042023100823