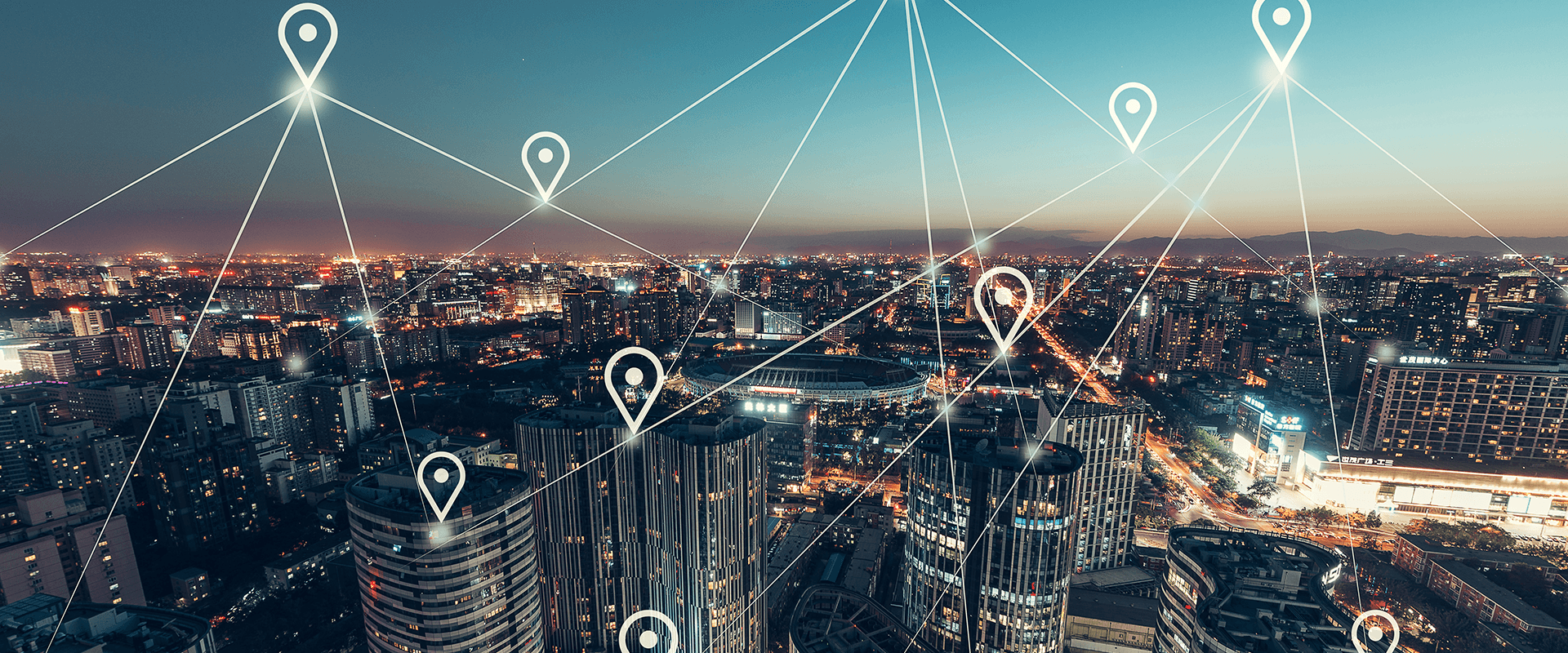
Delivering Complex Consumer Services: Driving Performance Through Data Analytics
- VOLUME XXI, ISSUE 24
- Executive Insights
Complex consumer service delivery models are under increasing pressure as competition grows, digital channels increase pricing scrutiny and customer expectations rise.
Many are looking to respond by better understanding customers’ needs, boosting efficiency and improving margins — but complex networks and scheduling dynamics make them notoriously difficult to accurately cost, analyze, optimize and manage.
Data analytics can help service delivery businesses to optimize operational costs while maintaining high levels of customer service, and secure competitive advantage.
Businesses must follow three key steps: understand their addressable cost base, identify “underlying” operating networks, and design and implement the optimal operating model.
Complex consumer service delivery models exist across a wide range of businesses, from central heating servicing, window installation and home security to roadside breakdown assistance and funeral provision. Like many other consumer businesses, they’re under increasing pressure as competition grows, digital channels increase pricing scrutiny, and customer expectations, shaped by market leaders such as Amazon, inexorably rise.
Service delivery businesses, such as British Gas and Sky, are looking to respond by better understanding and meeting customers’ needs, boosting efficiency, and improving margins. But these businesses are notoriously difficult to accurately cost, analyze, optimize and manage. They have complex networks and scheduling dynamics, relying on the right combination of high-value skills and equipment being in the right place at the right time, and multiple (mainly consumer-facing) steps in the delivery models make them hard to standardize.
The solution lies in the transformative power of combining data analytics with functional and business knowledge. Dramatic improvements in the cost effectiveness and accessibility of computing power and data have ushered in a new wave of analytical tools. While analytics has long been successfully applied to more standard problems (e.g., retail store planning and logistics), the latest wave of tools brings a new level of insight to the operational challenges of complex consumer service delivery models.
In this Executive Insights, L.E.K. Consulting outlines how consumer services businesses can use data analytics to optimize operational costs while maintaining high levels of customer service.
Many consumer services businesses lack the ability to measure the full cost to deliver specific services because those services leverage a variety of shared resources (e.g., people, vehicles, property and equipment). Such information is essential if improvements are to be made, and the task of tracking costs and defining key performance indicators is a fundamental area in which data analytics can help.
Although often straightforward to identify, the cost to deliver an individual service is often conflated or confused with other activities delivered from the same property or by the same vehicles or people. For example, in window installation, a single employee might advise on and handle customer queries, visit a customer’s home to measure the windows, and then visit again to fit the windows.
Unit costs can also vary by geography, and analytics can help businesses gain valuable insight into why differences occur (e.g., the number and geographic spread of consumers served by a set of shared resources), and identify the most economically attractive models nationwide.
A detailed picture of how services are delivered is necessary to assess efficiency. This requires the identification of “underlying” operating networks, which are the groups of resources frequently combined to deliver each service. Operating networks may not align with the business’s administrative structure, but examining them is the most effective way to assess performance and identify improvement opportunities, and should be the basis for KPIs and reporting.
Higher utilization levels are generally easier to achieve with larger resource pools, but organizations often fail to consolidate networks or maximize the benefits of scale that come from the networks they have. The reasons may be historical: The business grew through acquisition, networks grew organically, or the business uses several different operating models.
Applying analytics, such as standard network algorithms, to operational logs or records from scheduling systems enables operating networks to be identified, and facilitates an understanding of their measurable KPIs. Figure 1 illustrates an analysis of how resources are shared.
In this example, each color represents an operating network that contains a high level of shared resources. Here, we can make three observations: i) there are too many operating networks given the overall scale of resources; ii) resource-sharing is taking place between networks, compromising resource utilization (e.g., see operating networks 4&6) and iii) some networks have multiple centers, again leading to lower resource utilization (e.g., see operating network 3).
To improve performance, service delivery businesses must design a set of operating networks that contain the right quantity and combination of resources, operating from appropriately sized properties in the most effective locations. Upgrading the quality of local scheduling and management can also help increase efficiency, incentivize people more appropriately and provide the right level of support.
Detailed analysis of operating networks most commonly results in a reduced number in aggregate, eliminating subscale networks and realigning/redividing others (see Figure 2). This creates highly utilized assets, a workforce with centralized facilities, and a capex- and opex-efficient model.
An optimal network operating model must explicitly include goals for maintaining and improving service levels and minimizing the cost to achieve that, rather than only looking to minimize cost.
With the help of data analytics, organizations can iteratively test possible solutions to identify the optimal configuration; Figure 3 outlines the recommended approach. Constraints may include existing facilities, role definitions, working hours/breaks or vehicle capacities. Target customer service levels must be measurable and may include:
The computing power now available enables iterative testing of thousands of possible solutions against a set of customer jobs and estimated unit costs. The resulting target configuration requires review by operational experts to capture real-world operational knowledge — such as facility-specific constraints, access restrictions or local road conditions.
Once an optimal configuration has been agreed upon, delivery of the transformation plan will require new ways of working (see Case Study 1), including clear accountability and incentives for staff to deliver high-quality and cost-effective services.
Scheduling software is essential to roster resources for maximum efficiency, and new tools provide far greater solving power than manual systems can (see Case Study 2). Rostering systems should be linked to order-taking so that customers are nudged toward time slots that are operationally efficient, particularly when demand can be spread from peak to shoulder peak, avoiding a need for more capacity.
Data analytics represents an enormous opportunity for businesses with complex service delivery models because it can help reset a company’s expectations and change the nature of improvement goals from incremental to transformational. By following the steps outlined above, businesses can secure competitive advantage by significantly reducing costs, making marked improvements in efficiency and increasing the quality of customer service.
L.E.K. supported a client that had experienced rapid growth within its national network through acquisition, but had not reconsidered its original network configuration. As a result, the business was not effectively leveraging its scale.
However, the client had collected a rich data set on the multiple steps involved in delivering each customer job and the resources deployed. Use of data analytics to optimize the model in accordance with agreed-upon design principles resulted in a significant reduction in organizational complexity, decreasing the number of networks by approximately 40%.
Centrica, parent company of servicing brands such as British Gas and Dyno, is working with Microsoft to transform how it plans and dispatches work for its field engineers.
Mike Young, Centrica’s group Chief Information Officer, said the group would create “a seamless service” powered by artificial intelligence in the Microsoft Azure cloud platform. According to Young, this starts the next stage of the digital transformation, as Centrica builds cutting-edge intelligence into field operations in order to improve the service delivered to customers. Cindy Rose, Chief Executive of Microsoft U.K., states that this move “is a great example of how a large organization can build advanced intelligence into its operations to transform processes and empower employees to make better decisions.”
Further reading
Does Artificial Intelligence Mean the End of the Road for Travel Advisors?
Advanced Analytics: The Opportunity That Industrial Businesses Can’t Afford to Miss
How to Unlock Your Organization’s Data Analytics Potential