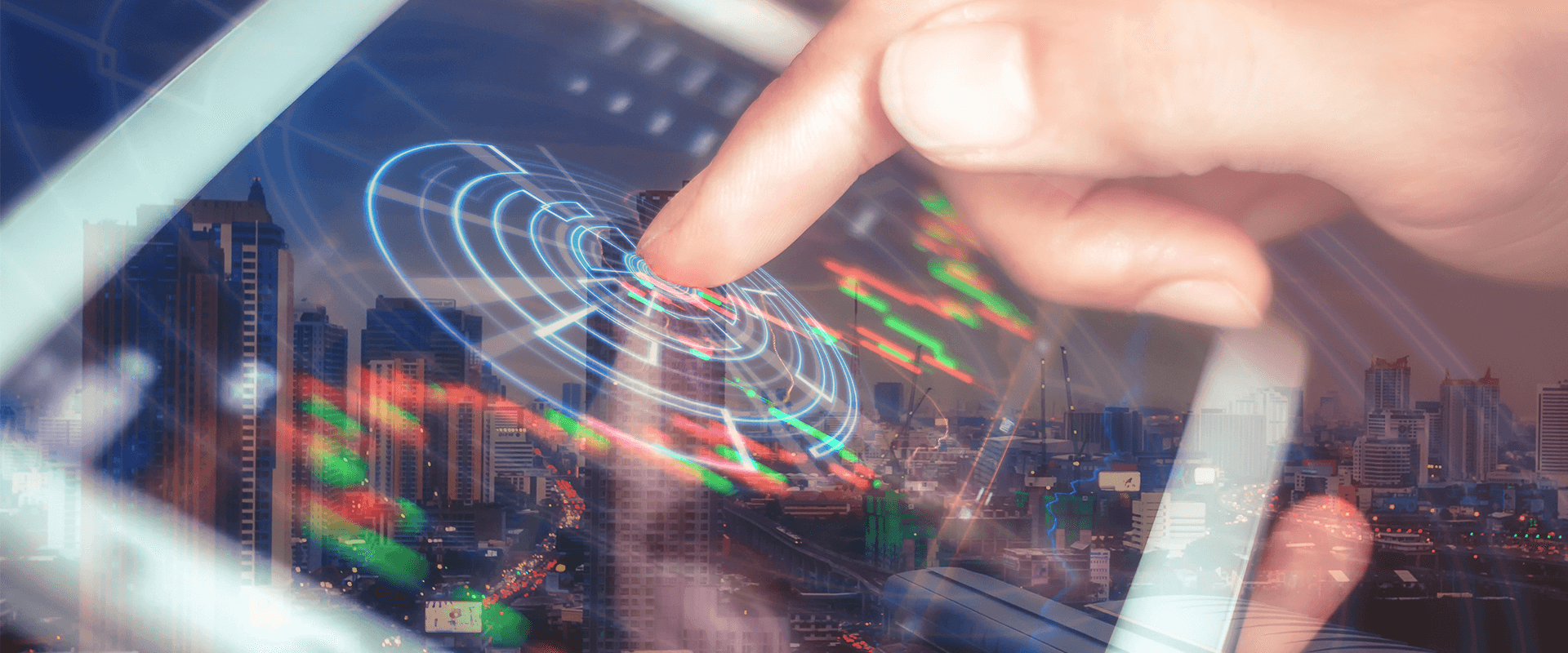
How to Unlock Your Organization’s Data Analytics Potential
- VOLUME XX, ISSUE 62
- Executive Insights
Most companies don’t need a small army of data scientists to put their data to good use – they need analyses that solve key commercial and operational problems.
The tools to do so are readily available and inexpensive, and many businesses already store commercially relevant data.
What is often missing is a clear strategy and operational model for using these capabilities in ways specific to the company’s business requirements.
L.E.K. examines how to get the most out of data analysis by building and integrating analytics capabilities throughout the organization.
Data and analytics professionals are at the center of the next big race for talent. In 2015, there was a surplus of people with data science skills. Now there’s a significant shortage1. By 2020, IBM expects demand for data and analytics talent to reach 2.7 million positions in the U.S. alone2.
The competition for talent will be especially intense for companies for whom advanced analytics forms a core part of their proposition — think e-commerce giants, hedge funds and complex system engineers. For them, a dedicated, in-house team of data specialists can be a necessity.
But the rest of us? Not so much. Consider the findings of a Rexer Analytics survey3 in which more than a third of data analytics professionals say their company never, or only sometimes, puts their analyses to use. This calls into question the practicality of funnelling analyses through centralized teams focused on big-picture challenges.
In our experience, most companies don’t need a small army of data scientists or bleeding-edge analytical techniques. What they do need are analyses that solve key commercial and operational problems. The good news is that the tools to do so are readily available — and relatively inexpensive. The same is true for processing power4. Meanwhile, the vast majority of companies already store (but don’t analyze) vast amounts of commercially-relevant data and are collecting it at a faster rate than ever before.
What’s missing, more often than not, is a clear strategy and operational model for using these capabilities in ways that are specific to the company’s business requirements. Any such effort depends on three basic components:
Together, these form a solid foundation for closing the gap between technical skills and commercial thinking so that businesses can extract value from analytics.
So how to get started? We suggest taking your cue from companies that have already started on this journey (see Figure 1).
Start small and demonstrate value. Aim for a single, key problem or an important (but limited) area of the business where analytics can have a valuable and immediate impact, then use the results to build credibility, excitement and momentum.
Brewing and beverage company SABMiller5 (now a division of Anheuser-Busch) took this track when they decided to make data and analysis available to their business units. To manage risk, they kept their initial investments modest. That way, they could readily abandon the failures while expanding the rollout of the tools and approaches that worked.
Focus on specific commercial problems. Tie analytics to the commercial and operational heart of your organization. (If it doesn’t address a core business need, analytics can be a hindrance more than a help.) While you’re at it, place the analytical capability as close as possible to those doing the commercial thinking.
Berlin-based Zalando6, for instance, empowered its business units with a self-service analytics infrastructure paired with embedded analysts focused on product development. The result? Teams ranging from Fashion Store to Logistics now can extract the data-informed insights they need to make smart decisions in line with the firm’s top business priorities.
Identify and empower evangelists. Don’t leave business users to figure out the commercial value of analytics on their own. Show it to them instead, via a network of advocates across the organization. Through these advocates, companies can proactively introduce the capabilities available to the business and provide expert support for those finding their way.
That’s what U.K. supermarket Sainsbury’s7 did when they created a new 60 strong internal team called the Humanalysts. The group’s mission? To identify data-driven opportunities for improvement — such as predicting shopper responses to new pricing strategies — and, along the way, make believers out of often skeptical business users.
Identify your analytical capabilities — both existing and potential. Many companies already have functions dedicated to strategy, financial planning or business insights, as well as individuals who use analysis to solve business problems. Build on these assets by empowering them with new capabilities. Special qualifications aren’t required — just the ability (and desire) to become data literate and proficient in the tools you select.
When Jaguar Land Rover8 discovered pockets of self-service analytics activity across its departments, the company began offering in-house analytics training. The first 60-seat course offering filled to capacity. By last year, an estimated 1,800 to 2,500 employees had become “citizen analysts” — business users who created their own analytics as part of their day-to-day work.
Establish a team to enable effective deployment. Building a small team of analytics professionals will help to ensure the effective deployment of analytics across the business. This team does not need to be comprised of data scientists — just individuals who are interested in and capable of developing appropriate analytics tools and techniques, and championing them across the organization (helping to provide internal training and advice to employees).
Build and rollout an appropriate toolkit. Encourage business users to tap into analytics with self-service tools that preclude the need to learn how to code. The most effective of these have built-in algorithms to navigate company data, create charts and dashboards, and deliver insights to different audiences.
Nike9 put together a tailored set of software tools for business intelligence, analysis and visualization — all with an aim to reduce technical barriers and bring insights to users in the business. The toolkit had to cover the full range of user requirements, from those just wanting key dashboards to those looking for as much data as possible to perform their own ‘exploratory’ analysis. All users benefit from a central team that provides the governance necessary to ensure a stable, secure and up-to-date environment.
Expand the toolkit. As more use cases are identified, it will be necessary to expand the analytic toolkit and the range of techniques used. It is important to retain the agile spirit and desire to experiment from earlier in the journey. Some of the new tools and techniques will work well, but a 100% success rate is unlikely. Assuming sensible, low cost experimentation, a two-thirds success rate on 15 opportunities is better than a 100% success rate on two.
Invest in people and processes. Now is the time to ensure sustainability. This can be achieved by investing in processes that are fit for the longer term and provide clarity on future career paths and opportunities for the core enabling team.
Leverage scale through standardization. As the level of adoption (and business benefit) grows, there are opportunities to leverage economies of scale. Examples of this include securing better licensing deals for software, increasing utilization of key analytic resources (both people and hardware) and maximizing efficiency (rather than expediency) of workflows as usage becomes more consistent.
Wherever you start your journey, keep this in mind: Democratizing analytics is an unavoidably iterative process. Every step requires a look back to ensure the appropriate controls, training and delivery mechanisms are in place and working the way they need to be. As Voltaire famously observed, with great power comes great responsibility.
It’s also a good idea to borrow liberally from those in similar situations to your own. Make generous use of relevant case studies. Prioritize the insights that generate commercial benefits. When reporting back within your organization, focus on output and impact rather than the underlying analytics. Inspire, and be inspired.
Finally, don’t give in to pressures to build great teams of scarce, highly-paid specialists without working out what makes sense for your business. There’s nothing so special about analytics that it must be kept from those most intimately familiar with the problems you need to solve, and who work for you already. Analytics should inherently empower anyone with the means to comprehend it. Put another way: Data analytics is for everyone — not just the few.
L.E.K. has long believed in democratizing analytics capability, and in the last few years we have evolved our own operating model to follow the principles described in this article. Our project teams are explicitly empowered to combine analytic and commercial insight so that the L.E.K. Data & Analytics team enables, rather than, replaces project teams. This small enabling team drives innovation in tools, data and techniques while providing expertise and guidance for project teams. Central to leveraging the wave of new self-service analytics applications has been the creation of a proprietary L.E.K. software toolkit for data gathering, management, analysis and visualization — all with the aim of accelerating the development of insights for our clients. Development of a set of standard analytical building blocks has accelerated solutions to common business problems and the team is responsible for light touch governance of data, processes and training on an ongoing basis.
Endnotes
1LinkedIn Workforce Report August 2018
2The Quant Crunch
3Data Science Survey
4Top cloud providers 2018
5Enabling Self-Service Analytics Across a Multi-National Brewing and Beverage Company
6Data For All: An Introduction to Product Analytics at Zalando
7Here’s How Sainsbury’s is Using Big Data to Drive Customer Experience
8Jaguar Land Rover turns to Tableau to give staff analytics dashboards that “just work”
9Self-service business intelligence
A version of this article was also published in Harvard Business Review.