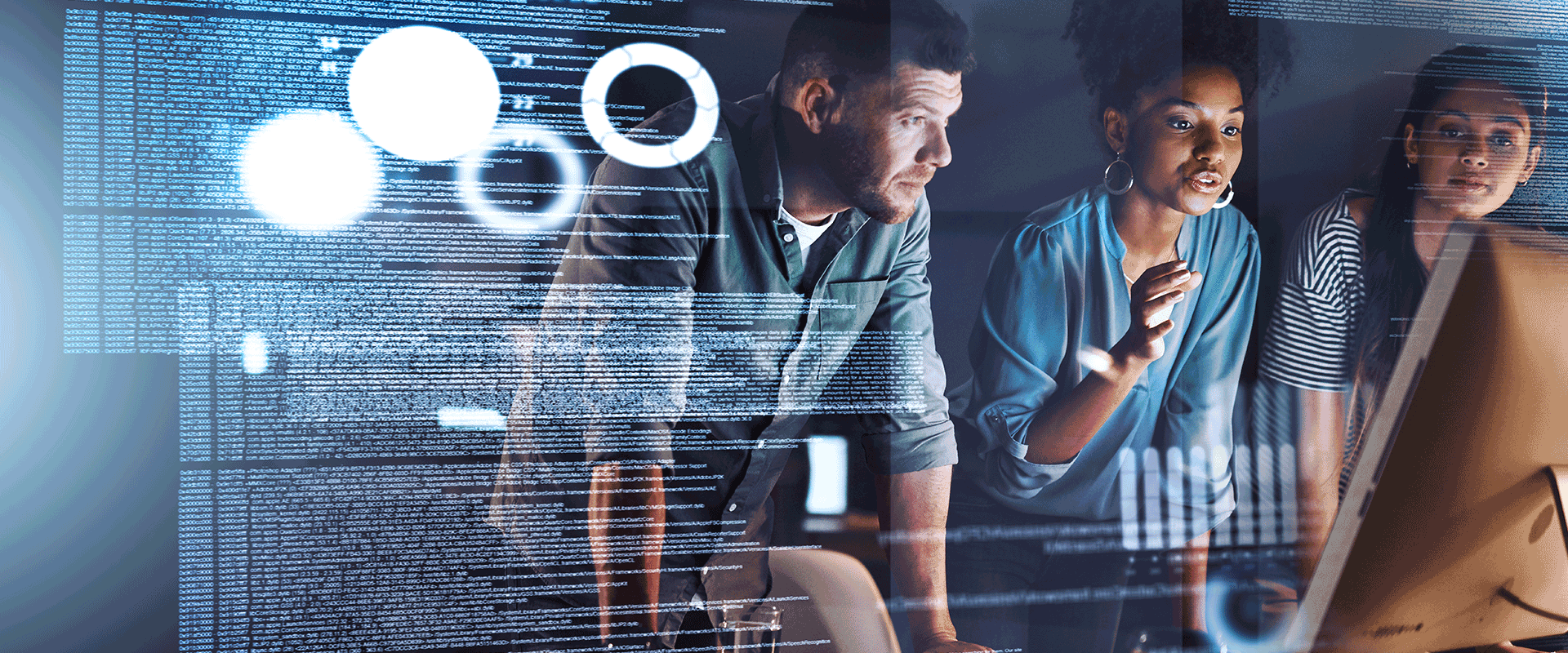
The Data-Driven Mindset
- Volume XXII, Issue 44
- Executive Insights
We all like to think data informs almost every key commercial decision a business makes. After all, the resources to do so are at hand: The amount of digital data is expected to reach 44 zettabytes in 2020, laptops have roughly 30 times more computing power than a decade ago and cloud computing usage is set to double in the next two years.
Still, something is holding businesses back. Too many continue to base their decisions on limited data, selectively interpreted to support management’s instincts or ambitions. It’s time for a new mindset — one that challenges old decision-making habits by continually asking:
The good news is that any organization can develop a data-driven mindset. The first step is to embrace a different way of thinking about the decisions the organization makes.
In a commercial organization, decisions tend to be one of several different types, characterized by their frequency and impact (see Figure 1). By thinking through the full taxonomy of decision types, companies can prioritize their data-driven decision-making and strike the appropriate balance of human judgment and computing power for each type of decision.
At one end of the spectrum are major strategic decisions. We define these as decisions that require significant financial investments or commitment and set the course of the business for the next few years, such as a high-profile acquisition or a corporate transformation effort. Such one-off, high-stakes decisions should be informed by analytical insights, where data can be used to enhance the decision-making of the organization’s commercial leaders.
At the other end are the many minor decisions that, somewhere in the business, a person or system makes every day. This could involve approving a credit application or giving a discount to a longtime customer. While small in individual impact, these decisions can accumulate to deliver a sizable punch over time — and data can increase both their speed and effectiveness. For instance, Netflix’s recommendation algorithm influences individual consumer choices, which collectively prevents the loss of an estimated $1 billion a year in revenue.
The decisions in between these two extremes are periodic ones that include some routine tasks that can be automated, such as combining or refreshing data. With decisions of the periodic type, the goal is to reserve human effort for the most sensitive and difficult matters. Consider Amazon’s “hands off the wheel” initiative, which automates routine processes such as demand forecasting and inventory deal negotiation so that highly paid employees can spend time on more valuable activities and intervene only when absolutely necessary.
So, what does a data-driven process look like? We recommend four steps to arrive at a decision, with each step requiring different capabilities.
1. Frame: Set decision criteria before seeing the evidence. This mitigates the cognitive bias that can occur when forming an opinion that the data inspires.
2. Access data: Obtain the data that addresses the question as framed. Usually this requires sourcing and cleaning for the first decision of each type. Data engineering capability is necessary to reliably make information available at scale.
3. Analyze and predict: Model decisions under a variety of assumptions and scenarios to reveal the range of potential outcomes. Because data is rarely perfect and typically reflects the past, the right data analytics capability is a must.
4. Decide: Visualize the data needed to make decisions, and share it with the right people at the right time.
In addition, we recommend two other steps to help evolve and enhance your decision-making capability on an ongoing basis.
5. Review and monitor: Track actual outcomes against predictions and incorporate lessons from experience to enhance future decisions and help demonstrate the value of data-driven decisions.
6. Evolve: Learn and change the way a decision is made given growing experience making that sort of decision. This could include automating and removing human involvement or pushing the decision down to less-senior people.
Although the process is important, it’s also incumbent on the decision-maker to appropriately use the analysis at his or her disposal. That means companies must have the following in place:
A decision architecture. To set this up, first identify the mosaic of decisions made across the organization, along with ways that data can improve these decisions. Next, prioritize the data enhancement opportunities and determine who — humans, bots or both — should make each decision going forward.
A data-driven culture. Make data literacy (the ability to correctly interpret information) an organizational imperative, starting at the top. As data-driven maturity increases, decision rights can extend further down the organization, reducing cost and turnaround time without impacting the probability of making the right decision.
For example, online retailer Stitch Fix combines machine learning (clothing suggestions based on purchases and personal information) with a human touch (such as pairing and accessory suggestions) to deliver personalization at scale. Tool hire company HSS Hire boosted its bottom line after rolling out enhanced analytics tools — supported by clear leadership and staff training — to enable smarter pricing, category management and fleet optimization.
Enhanced data and decision capabilities. Set the expectation that decision-makers are to embrace a data-driven mindset and acquire the skills and work methods to maximize the opportunity to make better decisions. Support them, and accelerate the spread of data literacy, with self-service analytics tools. Beyond that, add three types of capability: data engineering to reliably extract and create the data, data science to design the appropriate analytical models, and decision intelligence to make choices that reflect insights drawn from managerial and social sciences.
Google took this approach when it created decision intelligence capabilities dedicated to helping the business make better decisions — both by focusing on decision-making processes for specific issues and by training 20,000 Google employees to bridge the gap between data science and business needs.
The right data. To enable data-driven decisions, data must be relevant, reliable, repeatable and recognized as coming from an authoritative source. This requires data warehouses, lakes and pipelines. It also involves curation to guide potential users and help them interpret the data correctly.
For example, U.K. grocer Sainsbury’s recently announced plans to create an analytics platform aimed at empowering the entire organization, not just analysts, to use data to test hypotheses and make decisions.
A typical organization makes countless decisions every day. Whether it’s a one-off, multibillion-dollar investment or a day-today decision that helps make the business hum, each one can be enhanced through data, helping the organization be as efficient, effective and responsive as possible.
However, companies are commercial entities, and key decisions must be made by people with the relevant commercial context. The key to unlocking the value of data is to provide those decision-makers with the support they need to make the right decisions in a data-enabled way. Thus empowered, they can build a lasting competitive edge.