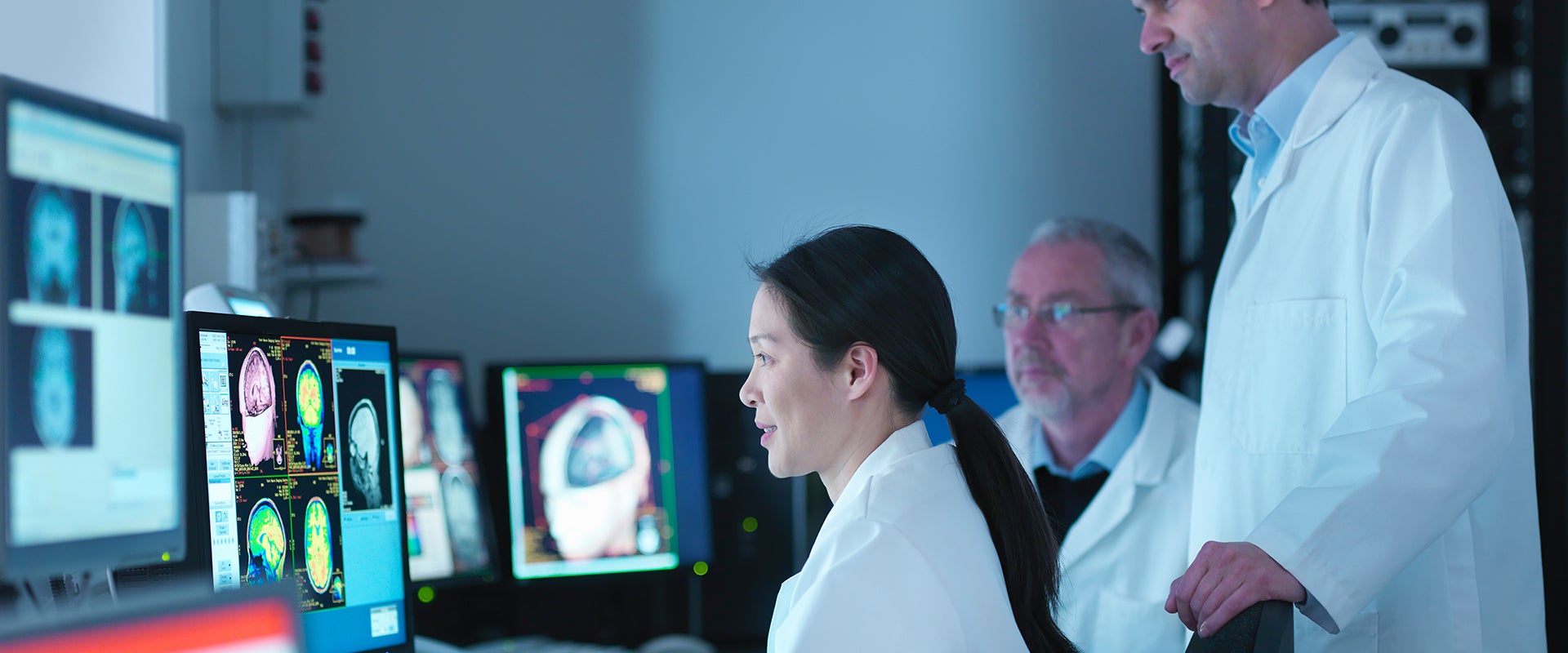
Artificial Intelligence: Six Challenges for the European Healthcare Sector
- VOLUME XX, ISSUE 59
- Executive Insights
AI applications are being developed across the healthcare sector.
Some of the most exciting innovations are in frontline care, where patients will benefit most directly.
AI applications can be used across the patient care pathway, from initial triage to monitoring ongoing treatment.
Adoption of the technology remains at an early stage, despite high levels of investment, research, and development and concept testing.
Before we see widespread use of AI, players must address six key barriers: develop effective partnerships; demonstrate clinical and economic benefits; create robust data sets; promote compliance with protection requirements; avoid future regulatory pitfalls; and develop liability management frameworks.
The revolutionary impact of AI on global healthcare could be felt in as little as the next five years. The hope is that lives will be saved, operational costs reduced and skills shortages eased, but alongside rapid advancements in AI capabilities come numerous challenges that must be addressed, especially concerning partnership development and data set creation.
Developments in AI applications are being made across the healthcare sector, including in back-office areas such as claims management and instrument maintenance programs, because of the relative lack of regulatory and registration hurdles. However, arguably the most exciting innovations are in frontline care, where patients will, over time, benefit most directly.
This Executive Insights focuses on some of the most promising developments in AI in the frontline of European healthcare. It also highlights the core challenges that healthcare organizations, from hospitals and their suppliers to insurers, need to be aware of and overcome before AI can be adopted on a scale wide enough to improve patient outcomes and system sustainability.
While there is no universal definition of AI, it broadly refers to systems that are able to function with a degree of autonomy and iteratively optimize their processes. The term “AI” can be applied to four major categories:
AI has the potential to offer benefits to the full range of healthcare stakeholders. With AI, patients will receive more rapid and accurate diagnoses than is possible with current systems, allowing for tailored treatment interventions with higher first-time success rates.
As diseases are managed more effectively, payers will see cost savings associated with improved patient outcomes, such as a reduction in the number of complications.
The overall efficiency of hospitals will be increased, as hospitals see a decrease in admissions and, as a result, a reduction in costs. In the U.S., Philadelphia’s Temple University Health System’s use of the tool Accolade is a powerful case study that demonstrates some of the early-stage wins that AI can bring: In two years the hospital has seen a 7% reduction in admissions and has saved nearly $10 million.
AI will also alleviate the significant and intensifying pressure of increasing staff shortages. The U.K.’s National Health Service (NHS), for example, has 45,000 clinical vacancies and a further 50,000 non-clinical open roles, and a similar lack of staff and capacity can be seen across Europe. Many of these positions are currently filled by temporary staff — a short-term fix that only serves to add further financial strain due to the higher costs associated with temporary employees. AI applications, such as those that conduct triage before patients arrive at a clinical facility, will give overstretched healthcare professionals greater leverage, allowing them to focus on interacting with patients on arrival.
The transformative capabilities of AI in healthcare are attracting substantial investment, with CB Insights reporting that AI deal activity across healthcare and life sciences has surpassed all other industries since 2013. Globally, healthcare AI companies raised $4.3 billion across more than 500 equity deals in this period. Funding levels and deal volume have grown significantly since 2013, with 2018 on track to significantly exceed 2017 levels (see Figure 1).
AI solutions have been developed that work with multiple data types, including patient-reported symptoms, biometric and biosensor data, imaging, and biomarker data (see Figures 2 & 3). These applications can be used across the patient care pathway, from initial triage to monitoring of ongoing treatment.
In spite of the high level of investment, research, and development and concept testing, adoption of AI applications remains at an early stage. Before we see the widespread use of the technology, there are six barriers that need to be overcome:
There is no doubt that AI is set to have a transformative impact on the healthcare sector. There is huge investment in the technology and a plethora of pilots are underway, but ensuring that the promise of AI can become a reality will not be straightforward. Many of the challenges that have slowed deployment of IT in healthcare environments will have to be overcome, and new ones will arise due to the dynamic characteristics of the technology involved. The nature of these challenges is becoming increasingly clear and emerging winners in the field are taking steps to actively address them as they ramp up their activity.