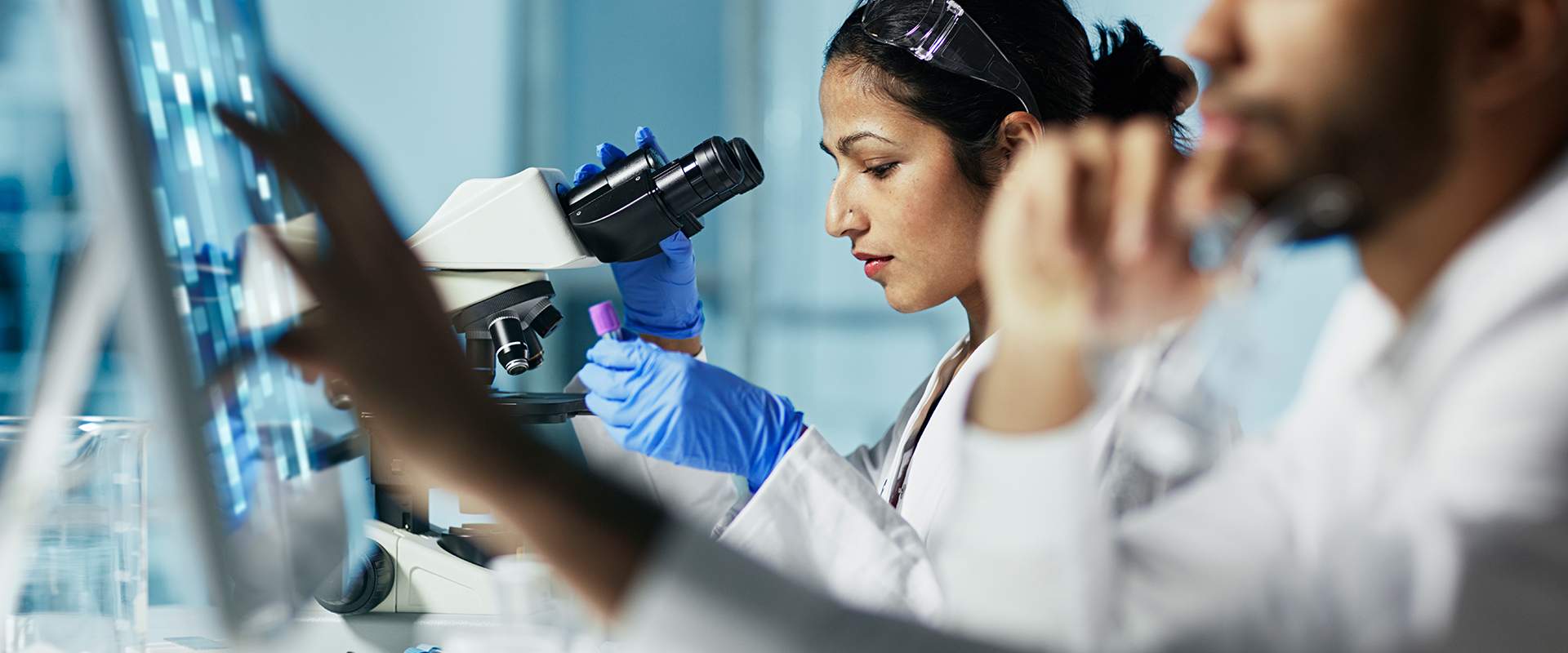
AI in Biopharma: Realizing the Promise
- Volume XXI, Issue 71
- Executive Insights
AI can help to address major drug development pain points, improving the quality of candidates, optimizing clinical trial design, reducing time to complete activities and reducing costs.
To date, biopharma has been cautious about adopting AI, with a number of real issues holding back progress.
To lower the barriers to adoption, biopharma companies should improve the collection and storage of internal data, ensure data sharing across divisions, have reasonable expectations and lead a change management effort.
To lower the barriers to adoption, AI companies should secure sufficient biopharma expertise, cater to their customers’ needs, work with high-quality data, deliver to promise and be patient (and not interpret customer conservatism as a lack of interest).
Artificial intelligence (AI) should enable biopharma companies to tackle the entrenched inefficiencies of the costly and time-consuming drug development process.
Drug approval rates, development costs, speed of delivery and patient compliance can all be improved with AI (see AI in Life Sciences: The Formula for Pharma Success Across the Drug Lifecycle). And yet, biopharma has been cautious in adopting AI solutions to date.
This reluctance to adopt reflects both the conservative nature of the industry and the very real issues that the industry must address before the promise of AI can be realized. In this Executive Insights, L.E.K. Consulting outlines the adoption barriers to AI in biopharma and identifies the key principles that biopharma and AI companies can follow to accelerate widespread adoption.
The development of new pharmaceuticals is a long and costly process (the largest 10 companies spend $67 billion on an annual basis), with less than 10% of clinical drug candidates reaching approval. According to market participants surveyed by L.E.K., specific challenges exist at each stage of the drug development process (see Figure 1):
Biopharma tends to be concerned with failures at the clinical stage, with approximately 70% of the total drug development costs incurred in the clinic. However, failure at this stage is often caused by suboptimal compounds, so enhancing earlier stages of drug development should result in higher success rates, highlighting the importance of addressing inefficiencies across the drug development spectrum.
There is widespread recognition among biopharma companies that the R&D process is inefficient and that this inefficiency will keep growing if left unaddressed. This dynamic is partly driven by the increasingly complex nature of the biology underpinning the discovery of new molecules and increasing regulatory requirements. Other contributing factors include the facts that most attainable targets or drugs have already been used and that defining clinical trials is getting harder as patient populations need to be defined more precisely to show the desired effect.
AI has the potential to help curb this trend through promising innovative solutions that address drug development pain points, focusing on:
In addition to streamlining steps across the R&D process, AI solutions also have the potential to redefine the process itself by cutting out steps altogether, for example by moving from target to lead directly, or bypassing the hit optimization process.
To date, biopharma has been more cautious in adopting AI than other industries, with a number of real issues holding back progress.
First, there are unclear “real-world” benefits. Publicly documented use cases demonstrate the efficacy of AI under specific conditions at specific stages of development, but they often fail to define the tangible impact on R&D cost, timelines or overall probability of success. AI companies have also only recently started to publicly share compelling evidence of their ability to address drug development pain points.
Second, due to the complex nature of machine learning, interpreting AI insights can be challenging. Biopharma stakeholders often find it hard to understand the decision-making process underpinning an AI solution, resulting in a lack of buy-in to findings.
Third, the availability of relevant and readily usable biopharma proprietary data is limited, and it often takes significant time to clean, identify and extract the data required to enable AI. Information often resides in multiple locations and across functions, and the amount of data relevant to a given target or drug may be limited or hard to access.
Fourth, biopharma has an internal resistance to change, and the industry is known for its cautious nature. This is particularly true of R&D teams, which tend to be wary of new approaches given how much is at stake if things go wrong. This may be exacerbated by a lack of experience within the industry, as few biopharma stakeholders have the relevant expertise in AI to fully appreciate the potential and limitations of its application in drug development.
Finally, the AI landscape is evolving rapidly. New companies are emerging, and existing organizations are continuously evolving their propositions and business models. It is challenging for biopharma to “pick winners” at this nascent stage of market development.
Despite the barriers to deployment, biopharma’s interest in AI remains high. The major players are either investing in AI technology or leading an AI-focused effort, alone or jointly with others, and face pressure not to fall behind the competition. AI providers are increasingly targeting their solutions to address specific industry needs, matching biopharma expectations and building trust by providing more precise, comprehensible and substantiated evidence.
To accelerate the advance of AI, biopharma companies and AI providers can follow a few key principles to lower barriers to adoption (see Figure 2).
After several years of experimentation and field-testing, it is time for biopharma players to plan the implementation of AI solutions more broadly in drug development. With the right focus from both AI companies and biopharma to address the barriers, L.E.K. expects that a significant proportion of R&D projects will have an AI component within the next five years.
Endnote:
1ADME = absorption, distribution, metabolism and excretion