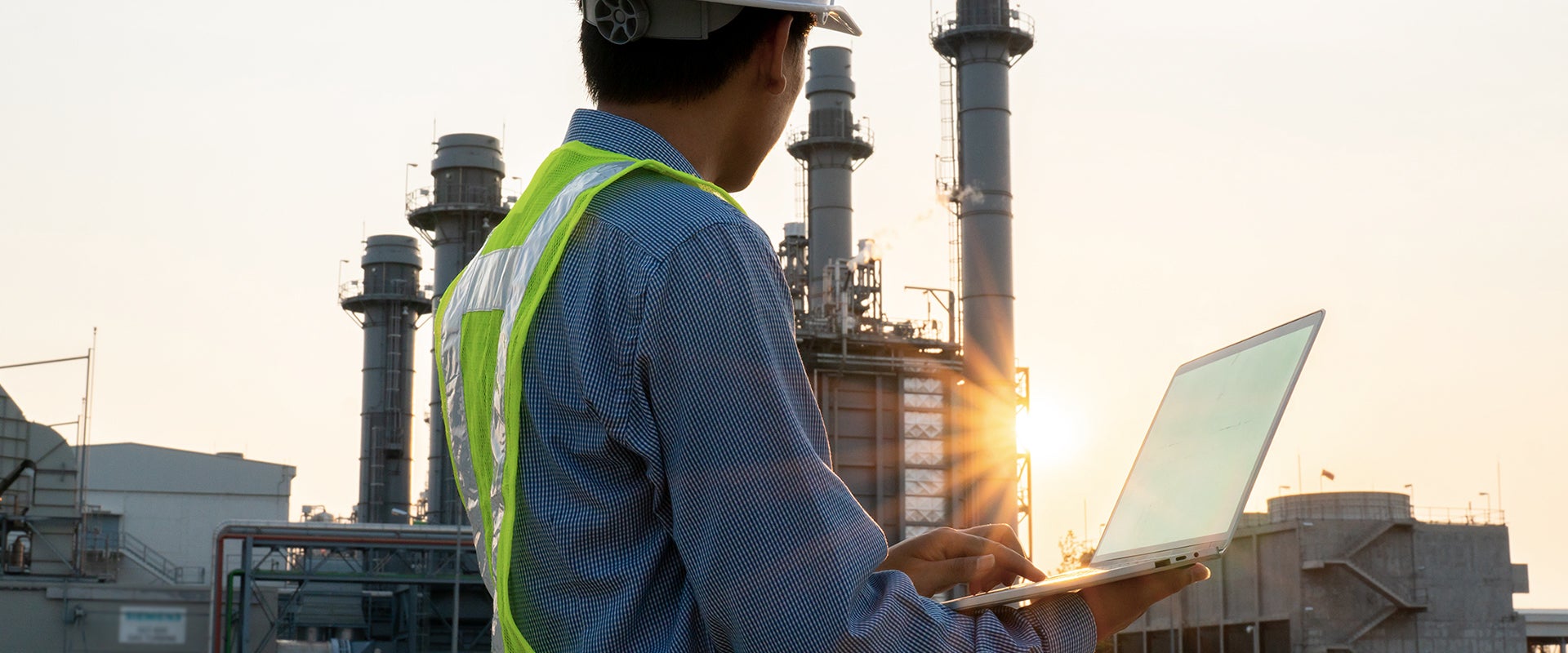
Best Practices in Advanced Data Science: Lessons for the Oil and Gas Industry
- Volume XXI, Issue 48
- Executive Insights
The oil and gas industry — which frequently operates at the cutting edge of science and engineering — stands to benefit considerably from data-driven analytics.
However, the industry is playing catch-up in the race to apply advanced data science to its most critical problems.
In our experience, companies should optimize activities in four key areas in order to reap the benefits of investments in digital technologies: good problem formulation, data readiness, expertise availability and organizational enablement.
In this Executive Insights, we provide examples of companies that have successfully deployed advanced analytics, and we offer manageable short-term initiatives that can then be broadened into more ambitious longer-term efforts.
Ask any oil and gas executive and that person, if being honest, may admit to a hidden concern: The industry is playing catch-up in the race to apply advanced data science — including machine learning and artificial intelligence (AI) — to its most critical problems. The perception that energy is lagging behind industries like retail or technology is understandable given the sheer visibility of AI solutions such as online recommendation engines or ride-sharing apps. But it is perhaps unfair, considering the enormous and complex challenges faced by oil and gas companies.
Unlike the tech industry, where “fail fast and fail often” is the mantra, or the retail industry, which has perfected techniques like A/B product testing, the cost of failure for the oil and gas industry is significant. This restricts the amount of experimentation companies can undertake and pushes them toward a focus on high-quality solutions. The complexity of engineering required to develop industry solutions means that AI approaches often need to be deployed into highly sophisticated situations, with multiple variables at play. What’s more, many activities, such as developing a field, happen relatively infrequently ― nearly 20,000 onshore wells are drilled in the United States per year. (Contrast that with 150 million Visa transactions per day, for example.) So obtaining data at the required scale for many algorithms, such as deep learning, which requires huge amounts of data, can be difficult. Finally, available data is often highly commercially valuable, so the incentives for sharing it across the industry can be low. Given all these factors, it is hardly surprising that the number of activities for which AI will be highly effective is relatively low compared with other industries. But when AI is applied to the appropriate areas, its impact is likely to be considerable (see Figure 1).
Clearly, industry players understand the potential of advanced data analytics and digital technologies in general, and the level of investment reflects this (see Figure 2).
The oil and gas industry — which frequently operates at the cutting edge of science and engineering — stands to benefit considerably from data-driven analytics, as that can be used to both supplement existing expertise and develop new approaches. For unconventionals, where today’s geophysics challenges are unique to the industry (e.g., parent-child well interference and flow diversion), data-driven approaches may further expedite new advances.
At L.E.K. Consulting, our experience has taught us that regardless of industry, companies need to optimize their activities in four key areas in order to reap the benefits of investments in digital technologies, and AI in particular. This is illustrated by the framework presented in Figure 3. Specifically, using AI to deliver sustained business value requires good problem formulation, data readiness, expertise availability and organizational enablement.
Not all problems can or should be addressed using advanced analytical techniques. Generally speaking, AI-driven solutions are appropriate for two broad classes of problems: 1) complex business decisions (both commercial and operational) that hinge on predictions inferred from historical data patterns, and 2) automation of commercial or operational processes with complex but discernible underlying patterns.
For example, GE determined that it could improve the effectiveness of its equipment maintenance by applying predictive algorithms to heat loss data. Heat loss monitoring enables anomalies in power plant equipment to be detected, indicating the need for maintenance. If anomalies are handled proactively, operators can avoid unplanned and costly downtime. However, certain critical components may not contain sensors and therefore cannot be easily monitored by service engineers. To address this, GE developed a heat-monitoring smartphone app called TITAN that uses an iPhone equipped with a thermal camera to provide noninvasive monitoring of these components. Thermal images can be classified as normal or anomalous based on engineers’ domain knowledge, providing a labeled dataset. This dataset informs an image recognition algorithm derived through machine learning, which can then proactively identify when equipment is in need of repairs. TITAN, which was based on an internal GE hackathon project, proved to be relatively low-cost to operate and was deployed to multiple power plants after initial tests yielded positive results.
AI and machine learning algorithms frequently require significant amounts of data to be effective, especially since both training and testing datasets are needed to effectively test and verify a model. In addition, the data must be of sufficient quality (both scrubbed and standardized) and granularity, and it must be appropriately representative of the phenomena being modeled.
BP was seeking to reduce fugitive emissions — gas emissions resulting from leaks or gases that are unintentionally released during industrial activities — that were significant in many of its mature fields in the continental United States. While BP engineers believed that machine learning algorithms could be very effective in reducing fugitive emissions, they still needed to obtain the data to develop and test appropriate models. However, outfitting all their wells with sensors to gather this data would be costly and, without a business case for doing so, hard to justify.
BP and its technology partners therefore came up with an inexpensive way to gather data and test their hypothesis. They fixed Android phones to a selection of beam pumps and then combined the data gathered with historical maintenance logs and weather recordings. This allowed them to test the algorithmic approach and prove the business case. Following this successful pilot, permanent sensors were installed that were able to yield large amounts of data on equipment telemetry and well conditions in real time.
Armed with a continuous influx of data, the algorithm now provides continual recommendations to engineers controlling the pumps remotely, allowing them to make the necessary changes to pressure and flow rates at each of the wells and minimize fugitive emissions.
Effective application of AI, of course, requires analytics expertise to ensure the right AI tools and technology are being implemented. But that is rarely enough. For the complex problems faced by the oil and gas industry, other capabilities are also critical, including domain experts to provide contextual understanding of the problem space, as well as individuals who are able to synthesize outcomes and then translate them into actionable insights — effectively closing the gap between technical skills and commercial understanding.
As part of its effort to optimize its overall energy portfolio, Exelon wanted to be able to accurately dispatch to the energy market excess power generated by its wind turbines. In order to do this, it needed a five-minute forecasting capability to predict when wind speed would change suddenly (so-called wind ramp events). The company, which uses wind turbines made by multiple manufacturers, was looking for an OEM-agnostic data aggregation and analytics solution, but did not have all the required capabilities and did not want the risk and cost of in-house development. It therefore decided to partner, ultimately turning to GE’s Renewables Data Science Team. Exelon provided the team with access to a year’s worth of turbine data to use in building and training machine learning models for wind ramp prediction.
The team first tested their approach on a sample of four wind turbines to prove the concept before rolling it out more widely. GE used their Predix industrial “Internet of Things” software within Exelon’s IT infrastructure for a purely software-based machine learning solution. The result was an increase in annual energy production of around 3%, and reduction in operating costs of 25%. The real-time forecasting model was also applied to longer-term forecasts, improving overall accuracy.
Scaling up AI solutions across multiple areas of the organization to achieve material performance impact requires a receptive organization. Senior leadership members need to be willing to step up and take ownership of the process. They must also facilitate overall organizational buy-in and empowerment in order to maximize use of the technology by personnel across all operational levels of the business. This should be supplemented by operations-level “evangelists” who take responsibility for sharing learnings and expertise across the organization.
Rio Tinto, in pursuing an ambitious “Mine of the Future” initiative, sought to combine its in-house mining and analytics expertise with the specialties of various partner companies, including Komatsu, Caterpillar and Amazon, to develop automation solutions for use in drilling, extraction and ore transportation.
To achieve these goals, Rio Tinto not only leveraged specific partner strengths, but also focused on designing supportive organizational structures. For example, it created a dedicated data science unit within a centralized innovation function that fostered dissemination of ideas across business units. It further promoted an open innovation culture by establishing an innovation lab and organizing hackathons.
Through this organizational setup, Rio Tinto succeeded in embedding cutting-edge automation as a central part of operations. Since 2014, it has been growing its use of automated haulage system (AHS) trucks, which now make up about 20% of the fleet at one of its major sites. The trucks lowered unit costs by 15%, and automated drills improved productivity by 10%. The company’s data science unit continues to deliver automation innovations such as smart crushers that can communicate with AHS trucks locally.
The examples described are just a few that show the benefits of deploying advanced analytics and machine learning. But getting started can be a daunting prospect, especially in an industry as complex as oil and gas. In our experience, it therefore makes sense to think in terms of manageable short-term initiatives that can then be broadened into more ambitious longer-term efforts.
It is clear that advanced data science applications have a place in the oil and gas industry, and their potential to yield tangible benefits is considerable. But unlike other industries, oil and gas faces challenges ranging from the enormous complexity of drilling operations and the high cost of failure to the difficulty of obtaining the quantity and quality of data required for the development and improvement of machine learning algorithms. Nevertheless, innovation has always been at the core of the oil and gas industry, and many companies are already finding creative ways to deploy data science solutions. Applying the lessons learned from these successes within the right framework will allow AI to be deployed successfully across the industry.
Editor’s note: This Executive Insights was adapted from the speech “Applied Data Science and Novel Measurements for Unconventional Development,” which was delivered by Stuart Robertson to the Society of Petroleum Engineers (SPE) workshop in May 2019.